Big Transfer Image Classification Model Quantization pipeline with NNCF#
This Jupyter notebook can be launched after a local installation only.
This tutorial demonstrates the Quantization of the Big Transfer Image Classification model, which is fine-tuned on the sub-set of ImageNet dataset with 10 class labels with NNCF. It uses BiT-M-R50x1/1 model, which is trained on ImageNet-21k. Big Transfer is a recipe for pre-training image classification models on large supervised datasets and efficiently fine-tuning them on any given target task. The recipe achieves excellent performance on a wide variety of tasks, even when using very few labeled examples from the target dataset. This tutorial uses OpenVINO backend for performing model quantization in NNCF.
Table of contents:
Installation Instructions#
This is a self-contained example that relies solely on its own code.
We recommend running the notebook in a virtual environment. You only need a Jupyter server to start. For details, please refer to Installation Guide.
import platform
%pip install -q "tensorflow-macos>=2.5; sys_platform == 'darwin' and platform_machine == 'arm64' and python_version > '3.8'" # macOS M1 and M2
%pip install -q "tensorflow>=2.5; sys_platform == 'darwin' and platform_machine != 'arm64' and python_version > '3.8'" # macOS x86
%pip install -q "tensorflow>=2.5; sys_platform != 'darwin' and python_version > '3.8'"
%pip install -q "openvino>=2024.0.0" "nncf>=2.7.0" "tensorflow-hub>=0.15.0" tf_keras
%pip install -q "scikit-learn>=1.3.2"
if platform.system() != "Windows":
%pip install -q "matplotlib>=3.4" "tensorflow_datasets>=4.9.0"
else:
%pip install -q "matplotlib>=3.4" "tensorflow_datasets>=4.9.0,<4.9.3"
import os
import numpy as np
from pathlib import Path
from openvino.runtime import Core
import openvino as ov
import nncf
import logging
from nncf.common.logging.logger import set_log_level
set_log_level(logging.ERROR)
from sklearn.metrics import accuracy_score
os.environ["TF_USE_LEGACY_KERAS"] = "1"
os.environ["TF_CPP_MIN_LOG_LEVEL"] = "2"
os.environ["TFHUB_CACHE_DIR"] = str(Path("./tfhub_modules").resolve())
import tensorflow as tf
import tensorflow_datasets as tfds
import tensorflow_hub as hub
tfds.core.utils.gcs_utils._is_gcs_disabled = True
os.environ["NO_GCE_CHECK"] = "true"
import requests
r = requests.get(
url="https://raw.githubusercontent.com/openvinotoolkit/openvino_notebooks/latest/utils/notebook_utils.py",
)
open("notebook_utils.py", "w").write(r.text)
core = Core()
tf.compat.v1.logging.set_verbosity(tf.compat.v1.logging.ERROR)
# For top 5 labels.
MAX_PREDS = 1
TRAINING_BATCH_SIZE = 128
BATCH_SIZE = 1
IMG_SIZE = (256, 256) # Default Imagenet image size
NUM_CLASSES = 10 # For Imagenette dataset
FINE_TUNING_STEPS = 1
LR = 1e-5
MEAN_RGB = (0.485 * 255, 0.456 * 255, 0.406 * 255) # From Imagenet dataset
STDDEV_RGB = (0.229 * 255, 0.224 * 255, 0.225 * 255) # From Imagenet dataset
Prepare Dataset#
datasets, datasets_info = tfds.load(
"imagenette/160px",
shuffle_files=True,
as_supervised=True,
with_info=True,
read_config=tfds.ReadConfig(shuffle_seed=0),
)
train_ds, validation_ds = datasets["train"], datasets["validation"]
def preprocessing(image, label):
image = tf.image.resize(image, IMG_SIZE)
image = tf.cast(image, tf.float32) / 255.0
label = tf.one_hot(label, NUM_CLASSES)
return image, label
train_dataset = train_ds.map(preprocessing, num_parallel_calls=tf.data.experimental.AUTOTUNE).batch(TRAINING_BATCH_SIZE).prefetch(tf.data.experimental.AUTOTUNE)
validation_dataset = (
validation_ds.map(preprocessing, num_parallel_calls=tf.data.experimental.AUTOTUNE).batch(TRAINING_BATCH_SIZE).prefetch(tf.data.experimental.AUTOTUNE)
)
# Class labels dictionary with imagenette sample names and classes
lbl_dict = dict(
n01440764="tench",
n02102040="English springer",
n02979186="cassette player",
n03000684="chain saw",
n03028079="church",
n03394916="French horn",
n03417042="garbage truck",
n03425413="gas pump",
n03445777="golf ball",
n03888257="parachute",
)
# Imagenette samples name index
class_idx_dict = [
"n01440764",
"n02102040",
"n02979186",
"n03000684",
"n03028079",
"n03394916",
"n03417042",
"n03425413",
"n03445777",
"n03888257",
]
def label_func(key):
return lbl_dict[key]
Plotting data samples#
import matplotlib.pyplot as plt
# Get the class labels from the dataset info
class_labels = datasets_info.features["label"].names
# Display labels along with the examples
num_examples_to_display = 4
fig, axes = plt.subplots(nrows=1, ncols=num_examples_to_display, figsize=(10, 5))
for i, (image, label_index) in enumerate(train_ds.take(num_examples_to_display)):
label_name = class_labels[label_index.numpy()]
axes[i].imshow(image.numpy())
axes[i].set_title(f"{label_func(label_name)}")
axes[i].axis("off")
plt.tight_layout()
plt.show()
2024-01-26 10:40:54.747316: W tensorflow/core/kernels/data/cache_dataset_ops.cc:854] The calling iterator did not fully read the dataset being cached. In order to avoid unexpected truncation of the dataset, the partially cached contents of the dataset will be discarded. This can happen if you have an input pipeline similar to dataset.cache().take(k).repeat(). You should use dataset.take(k).cache().repeat() instead.
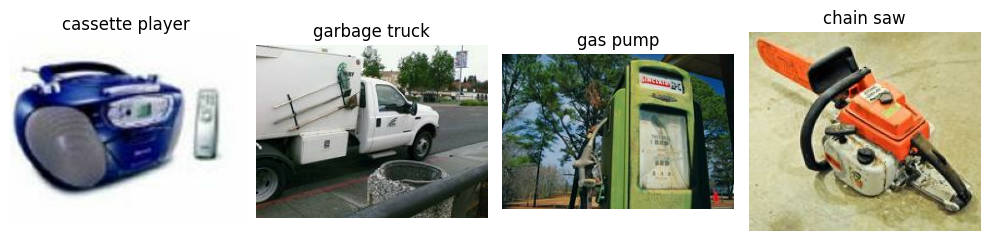
# Get the class labels from the dataset info
class_labels = datasets_info.features["label"].names
# Display labels along with the examples
num_examples_to_display = 4
fig, axes = plt.subplots(nrows=1, ncols=num_examples_to_display, figsize=(10, 5))
for i, (image, label_index) in enumerate(validation_ds.take(num_examples_to_display)):
label_name = class_labels[label_index.numpy()]
axes[i].imshow(image.numpy())
axes[i].set_title(f"{label_func(label_name)}")
axes[i].axis("off")
plt.tight_layout()
plt.show()
2024-01-26 10:40:57.011386: W tensorflow/core/kernels/data/cache_dataset_ops.cc:854] The calling iterator did not fully read the dataset being cached. In order to avoid unexpected truncation of the dataset, the partially cached contents of the dataset will be discarded. This can happen if you have an input pipeline similar to dataset.cache().take(k).repeat(). You should use dataset.take(k).cache().repeat() instead.
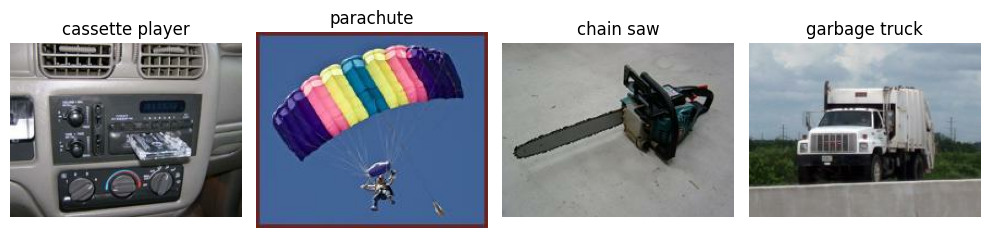
Model Fine-tuning#
# Load the Big Transfer model
bit_model_url = "https://www.kaggle.com/models/google/bit/frameworks/TensorFlow2/variations/m-r50x1/versions/1"
bit_m = hub.KerasLayer(bit_model_url, trainable=True)
tf_model_dir = Path("bit_tf_model")
# Customize the model for the new task
model = tf.keras.Sequential([bit_m, tf.keras.layers.Dense(NUM_CLASSES, activation="softmax")])
# Compile the model
model.compile(
optimizer=tf.keras.optimizers.Adam(learning_rate=LR),
loss="categorical_crossentropy",
metrics=["accuracy"],
)
# Fine-tune the model
model.fit(
train_dataset.take(3000),
epochs=FINE_TUNING_STEPS,
validation_data=validation_dataset.take(1000),
)
model.save(tf_model_dir, save_format="tf")
101/101 [==============================] - 472s 4s/step - loss: 0.4904 - accuracy: 0.8806 - val_loss: 0.0810 - val_accuracy: 0.9840
Perform model optimization (IR) step#
ir_path = Path("bit_ov_model/bit_m_r50x1_1.xml")
if not ir_path.exists():
print("Initiating model optimization..!!!")
ov_model = ov.convert_model("./bit_tf_model")
ov.save_model(ov_model, ir_path)
else:
print(f"IR model {ir_path} already exists.")
Initiating model optimization..!!!
Compute accuracy of the TF model#
tf_model = tf.keras.models.load_model(tf_model_dir)
tf_predictions = []
gt_label = []
for _, label in validation_dataset:
for cls_label in label:
l_list = cls_label.numpy().tolist()
gt_label.append(l_list.index(1))
for img_batch, label_batch in validation_dataset:
tf_result_batch = tf_model.predict(img_batch, verbose=0)
for i in range(len(img_batch)):
tf_result = tf_result_batch[i]
tf_result = tf.reshape(tf_result, [-1])
top5_label_idx = np.argsort(tf_result)[-MAX_PREDS::][::-1]
tf_predictions.append(top5_label_idx)
# Convert the lists to NumPy arrays for accuracy calculation
tf_predictions = np.array(tf_predictions)
gt_label = np.array(gt_label)
tf_acc_score = accuracy_score(tf_predictions, gt_label)
2024-01-26 10:51:24.539777: W tensorflow/core/common_runtime/graph_constructor.cc:839] Node 're_lu_48/PartitionedCall' has 1 outputs but the _output_shapes attribute specifies shapes for 2 outputs. Output shapes may be inaccurate.
2024-01-26 10:51:24.539856: W tensorflow/core/common_runtime/graph_constructor.cc:839] Node 'global_average_pooling2d/PartitionedCall' has 1 outputs but the _output_shapes attribute specifies shapes for 3 outputs. Output shapes may be inaccurate.
Compute accuracy of the OpenVINO model#
Select device for inference:
from notebook_utils import device_widget
device = device_widget()
device
core = ov.Core()
ov_fp32_model = core.read_model(ir_path)
ov_fp32_model.reshape([1, IMG_SIZE[0], IMG_SIZE[1], 3])
# Target device set to CPU (Other options Ex: AUTO/GPU/dGPU/)
compiled_model = ov.compile_model(ov_fp32_model, device.value)
output = compiled_model.outputs[0]
ov_predictions = []
for img_batch, _ in validation_dataset:
for image in img_batch:
image = tf.expand_dims(image, axis=0)
pred = compiled_model(image)[output]
ov_result = tf.reshape(pred, [-1])
top_label_idx = np.argsort(ov_result)[-MAX_PREDS::][::-1]
ov_predictions.append(top_label_idx)
fp32_acc_score = accuracy_score(ov_predictions, gt_label)
Quantize OpenVINO model using NNCF#
Model Quantization using NNCF
Preprocessing and preparing validation samples for NNCF calibration
Perform NNCF Quantization on OpenVINO FP32 model
Serialize Quantized OpenVINO INT8 model
def nncf_preprocessing(image, label):
image = tf.image.resize(image, IMG_SIZE)
image = image - MEAN_RGB
image = image / STDDEV_RGB
return image
int8_ir_path = Path("bit_ov_int8_model/bit_m_r50x1_1_ov_int8.xml")
val_ds = validation_ds.map(nncf_preprocessing, num_parallel_calls=tf.data.experimental.AUTOTUNE).batch(1).prefetch(tf.data.experimental.AUTOTUNE)
calibration_dataset = nncf.Dataset(val_ds)
ov_fp32_model = core.read_model(ir_path)
ov_int8_model = nncf.quantize(ov_fp32_model, calibration_dataset, fast_bias_correction=False)
ov.save_model(ov_int8_model, int8_ir_path)
Output()
Output()
Compute accuracy of the quantized model#
nncf_quantized_model = core.read_model(int8_ir_path)
nncf_quantized_model.reshape([1, IMG_SIZE[0], IMG_SIZE[1], 3])
# Target device set to CPU by default
compiled_model = ov.compile_model(nncf_quantized_model, device.value)
output = compiled_model.outputs[0]
ov_predictions = []
inp_tensor = nncf_quantized_model.inputs[0]
out_tensor = nncf_quantized_model.outputs[0]
for img_batch, _ in validation_dataset:
for image in img_batch:
image = tf.expand_dims(image, axis=0)
pred = compiled_model(image)[output]
ov_result = tf.reshape(pred, [-1])
top_label_idx = np.argsort(ov_result)[-MAX_PREDS::][::-1]
ov_predictions.append(top_label_idx)
int8_acc_score = accuracy_score(ov_predictions, gt_label)
Compare FP32 and INT8 accuracy#
print(f"Accuracy of the tensorflow model (fp32): {tf_acc_score * 100: .2f}%")
print(f"Accuracy of the OpenVINO optimized model (fp32): {fp32_acc_score * 100: .2f}%")
print(f"Accuracy of the OpenVINO quantized model (int8): {int8_acc_score * 100: .2f}%")
accuracy_drop = fp32_acc_score - int8_acc_score
print(f"Accuracy drop between OV FP32 and INT8 model: {accuracy_drop * 100:.1f}% ")
Accuracy of the tensorflow model (fp32): 98.40%
Accuracy of the OpenVINO optimized model (fp32): 98.40%
Accuracy of the OpenVINO quantized model (int8): 98.00%
Accuracy drop between OV FP32 and INT8 model: 0.4%
Compare inference results on one picture#
# Accessing validation sample
sample_idx = 50
vds = datasets["validation"]
if len(vds) > sample_idx:
sample = vds.take(sample_idx + 1).skip(sample_idx).as_numpy_iterator().next()
else:
print("Dataset does not have enough samples...!!!")
# Image data
sample_data = sample[0]
# Label info
sample_label = sample[1]
# Image data pre-processing
image = tf.image.resize(sample_data, IMG_SIZE)
image = tf.expand_dims(image, axis=0)
image = tf.cast(image, tf.float32) / 255.0
# OpenVINO inference
def ov_inference(model: ov.Model, image) -> str:
compiled_model = ov.compile_model(model, device.value)
output = compiled_model.outputs[0]
pred = compiled_model(image)[output]
ov_result = tf.reshape(pred, [-1])
pred_label = np.argsort(ov_result)[-MAX_PREDS::][::-1]
return pred_label
# OpenVINO FP32 model
ov_fp32_model = core.read_model(ir_path)
ov_fp32_model.reshape([1, IMG_SIZE[0], IMG_SIZE[1], 3])
# OpenVINO INT8 model
ov_int8_model = core.read_model(int8_ir_path)
ov_int8_model.reshape([1, IMG_SIZE[0], IMG_SIZE[1], 3])
# OpenVINO FP32 model inference
ov_fp32_pred_label = ov_inference(ov_fp32_model, image)
print(f"Predicted label for the sample picture by float (fp32) model: {label_func(class_idx_dict[int(ov_fp32_pred_label)])}\n")
# OpenVINO FP32 model inference
ov_int8_pred_label = ov_inference(ov_int8_model, image)
print(f"Predicted label for the sample picture by qunatized (int8) model: {label_func(class_idx_dict[int(ov_int8_pred_label)])}\n")
# Plotting the image sample with ground truth
plt.figure()
plt.imshow(sample_data)
plt.title(f"Ground truth: {label_func(class_idx_dict[sample_label])}")
plt.axis("off")
plt.show()
Predicted label for the sample picture by float (fp32) model: gas pump
Predicted label for the sample picture by qunatized (int8) model: gas pump
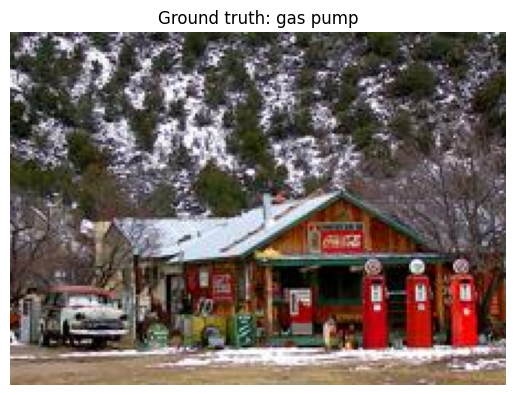