Stable Diffusion with KerasCV and OpenVINO#
This Jupyter notebook can be launched after a local installation only.
Warning
Important note: This notebook requires python >= 3.9. Please make sure that your environment fulfill to this requirement before running it
Stable Diffusion is a powerful, open-source text-to-image generation model. There are multiple implementations of this pipeline in different frameworks. Previously, we already considered how to convert and optimize PyTorch Stable Diffusion using HuggingFace Diffusers library. In this tutorial, we consider how to convert and run Stable Diffusion from KerasCV that employs graph mode execution, which enhances performance by leveraging graph optimization and enabling parallelism and in the same time maintains a user-friendly interface for image generation. An additional part demonstrates how to run optimization with NNCF to speed up pipeline.
Table of contents:
This is a self-contained example that relies solely on its own code.
We recommend running the notebook in a virtual environment. You only need a Jupyter server to start. For details, please refer to Installation Guide.
Prerequisites#
import platform
%pip install -q "tensorflow-macos>=2.15; sys_platform == 'darwin' and platform_machine == 'arm64' and python_version > '3.8'" # macOS M1 and M2
%pip install -q "tensorflow>=2.15; sys_platform == 'darwin' and platform_machine != 'arm64' and python_version > '3.8'" # macOS x86
%pip install -q "tensorflow>=2.15; sys_platform != 'darwin' and python_version > '3.8'"
%pip install -q keras-cv tf_keras numpy "openvino>=2024.1.0" "gradio>=4.19" datasets "nncf>=2.10.0"
if platform.system() != "Windows":
%pip install -q "matplotlib>=3.4"
else:
%pip install -q "matplotlib>=3.4,<3.7"
Convert Stable Diffusion Pipeline models to OpenVINO#
Stable Diffusion consists of three parts:
A text encoder, which turns your prompt into a latent vector.
A diffusion model, which repeatedly “denoises” a 64x64 latent image patch.
A decoder, which turns the final 64x64 latent patch into a higher-resolution 512x512 image.
workflow-diagram#
Let us convert each model to OpenVINO format.
Import required modules and set constants
import os
os.environ["TF_USE_LEGACY_KERAS"] = "1"
import keras_cv
import openvino as ov
import numpy as np
from pathlib import Path
import requests
# Fetch `notebook_utils` module
r = requests.get(url="https://raw.githubusercontent.com/openvinotoolkit/openvino_notebooks/latest/utils/notebook_utils.py")
open("notebook_utils.py", "w").write(r.text)
from notebook_utils import download_file
IMAGE_WIDTH = 512
IMAGE_HEIGHT = 512
BATCH_SIZE = 1
MAX_PROMPT_LENGTH = 77
OV_TEXT_ENCODER_MODEL_PATH = Path("models/ov_text_encoder_model.xml")
OV_DIFFUSION_MODEL_PATH = Path("models/ov_diffusion_model.xml")
OV_DECODER_MODEL_PATH = Path("models/ov_decoder_model.xml")
Create KerasCV Stable Diffusion pipeline
pipeline = keras_cv.models.StableDiffusion(img_width=IMAGE_WIDTH, img_height=IMAGE_HEIGHT)
Convert text encoder#
Text encoder has 2 inputs: tokens
and positions
. Specify inputs
shapes and provide example data for model tracing.
text_encoder_input = {
"tokens": (BATCH_SIZE, MAX_PROMPT_LENGTH),
"positions": (BATCH_SIZE, MAX_PROMPT_LENGTH),
}
text_encoder_example_input = (
np.random.randint(len(pipeline.tokenizer.vocab), size=(1, MAX_PROMPT_LENGTH)),
np.expand_dims(np.arange(MAX_PROMPT_LENGTH), axis=0),
)
ov_text_encoder = ov.convert_model(
pipeline.text_encoder,
example_input=text_encoder_example_input,
input=text_encoder_input,
)
ov.save_model(ov_text_encoder, OV_TEXT_ENCODER_MODEL_PATH)
del ov_text_encoder
Convert diffusion model#
Diffusion model has 3 inputs latent
, timestep_embedding
and
context
. Specify inputs shapes and provide example data for model
tracing.
diffusion_model_input = {
"latent": [BATCH_SIZE, pipeline.img_height // 8, pipeline.img_width // 8, 4],
"timestep_embedding": [BATCH_SIZE, 320],
"context": [BATCH_SIZE, MAX_PROMPT_LENGTH, 768],
}
diffusion_model_example_input = (
np.random.random(size=(1, pipeline.img_height // 8, pipeline.img_width // 8, 4)),
np.random.random(size=(1, 320)),
np.random.random(size=(1, MAX_PROMPT_LENGTH, 768)),
)
ov_diffusion_model = ov.convert_model(
pipeline.diffusion_model,
input=diffusion_model_input,
example_input=diffusion_model_example_input,
)
ov.save_model(ov_diffusion_model, OV_DIFFUSION_MODEL_PATH)
del ov_diffusion_model
Convert decoder#
Decoder has 1 input for image latents. Specify input shapes and provide example data for model tracing.
decoder_input = [BATCH_SIZE, pipeline.img_height // 8, pipeline.img_width // 8, 4]
decoder_example_input = np.random.random(size=(1, pipeline.img_height // 8, pipeline.img_width // 8, 4))
ov_decoder = ov.convert_model(pipeline.decoder, input=decoder_input, example_input=decoder_example_input)
ov.save_model(ov_decoder, OV_DECODER_MODEL_PATH)
del ov_decoder
# free memory
import gc
del pipeline
gc.collect();
Stable Diffusion Pipeline with OpenVINO#
Let’s take KerasCV pipeline implementation and replace original models with OpenVINO ones.
"""
Credits:
- Original implementation:
https://github.com/CompVis/stable-diffusion
- Initial TF/Keras port:
https://github.com/divamgupta/stable-diffusion-tensorflow
- Keras CV implementation:
https://github.com/keras-team/keras-cv/tree/master/keras_cv/models/stable_diffusion
"""
import math
import tf_keras as keras
import numpy as np
import tensorflow as tf
from pathlib import Path
from keras_cv.models.stable_diffusion import SimpleTokenizer
if not Path("./constants.py").exists():
download_file(url="https://raw.githubusercontent.com/openvinotoolkit/openvino_notebooks/latest/notebooks/stable-diffusion-keras-cv/constants.py")
from constants import UNCONDITIONAL_TOKENS, ALPHAS_CUMPROD
class StableDiffusion:
def __init__(self, text_encoder, diffusion_model, decoder):
# UNet requires multiples of 2**7 = 128
img_height = round(IMAGE_HEIGHT / 128) * 128
img_width = round(IMAGE_WIDTH / 128) * 128
self.img_height = img_height
self.img_width = img_width
self._tokenizer = None
self._text_encoder = text_encoder
self._diffusion_model = diffusion_model
self._decoder = decoder
print(
"By using this model checkpoint, you acknowledge that its usage is "
"subject to the terms of the CreativeML Open RAIL-M license at "
"https://raw.githubusercontent.com/CompVis/stable-diffusion/main/LICENSE"
)
def text_to_image(
self,
prompt,
negative_prompt=None,
num_steps=50,
unconditional_guidance_scale=7.5,
seed=None,
):
encoded_text = self.encode_text(prompt)
return self._generate_image(
encoded_text,
negative_prompt=negative_prompt,
batch_size=BATCH_SIZE,
num_steps=num_steps,
unconditional_guidance_scale=unconditional_guidance_scale,
seed=seed,
)
def encode_text(self, prompt):
# Tokenize prompt (i.e. starting context)
inputs = self.tokenizer.encode(prompt)
if len(inputs) > MAX_PROMPT_LENGTH:
raise ValueError(f"Prompt is too long (should be <= {MAX_PROMPT_LENGTH} tokens)")
phrase = inputs + [49407] * (MAX_PROMPT_LENGTH - len(inputs))
phrase = tf.convert_to_tensor([phrase], dtype="int32")
return self.text_encoder({"tokens": phrase, "positions": self._get_pos_ids()})
def text_encoder(self, args):
return self._call_ov_model(self._text_encoder, args)
def diffusion_model(self, args):
return self._call_ov_model(self._diffusion_model, args)
def decoder(self, args):
return self._call_ov_model(self._decoder, args)
def _generate_image(
self,
encoded_text,
negative_prompt=None,
batch_size=BATCH_SIZE,
num_steps=50,
unconditional_guidance_scale=7.5,
diffusion_noise=None,
seed=None,
):
if diffusion_noise is not None and seed is not None:
raise ValueError(
"`diffusion_noise` and `seed` should not both be passed to "
"`generate_image`. `seed` is only used to generate diffusion "
"noise when it's not already user-specified."
)
context = self._expand_tensor(encoded_text, batch_size)
if negative_prompt is None:
unconditional_context = np.repeat(self._get_unconditional_context(), batch_size, axis=0)
else:
unconditional_context = self.encode_text(negative_prompt)
unconditional_context = self._expand_tensor(unconditional_context, batch_size)
if diffusion_noise is not None:
diffusion_noise = np.squeeze(diffusion_noise)
if len(np.shape(diffusion_noise)) == 3:
diffusion_noise = np.repeat(np.expand_dims(diffusion_noise, axis=0), batch_size, axis=0)
latent = diffusion_noise
else:
latent = self._get_initial_diffusion_noise(batch_size, seed)
# Iterative reverse diffusion stage
num_timesteps = 1000
ratio = (num_timesteps - 1) / (num_steps - 1)
timesteps = (np.arange(0, num_steps) * ratio).round().astype(np.int64)
alphas, alphas_prev = self._get_initial_alphas(timesteps)
progbar = keras.utils.Progbar(len(timesteps))
iteration = 0
for index, timestep in list(enumerate(timesteps))[::-1]:
latent_prev = latent # Set aside the previous latent vector
t_emb = self._get_timestep_embedding(timestep, batch_size)
unconditional_latent = self.diffusion_model(
{
"latent": latent,
"timestep_embedding": t_emb,
"context": unconditional_context,
}
)
latent = self.diffusion_model(
{
"latent": latent,
"timestep_embedding": t_emb,
"context": context,
}
)
latent = np.array(unconditional_latent + unconditional_guidance_scale * (latent - unconditional_latent))
a_t, a_prev = alphas[index], alphas_prev[index]
# Keras backend array need to cast explicitly
target_dtype = latent_prev.dtype
latent = np.array(latent, target_dtype)
pred_x0 = (latent_prev - math.sqrt(1 - a_t) * latent) / math.sqrt(a_t)
latent = np.array(latent) * math.sqrt(1.0 - a_prev) + math.sqrt(a_prev) * pred_x0
iteration += 1
progbar.update(iteration)
# Decoding stage
decoded = self.decoder(latent)
decoded = ((decoded + 1) / 2) * 255
return np.clip(decoded, 0, 255).astype("uint8")
def _get_unconditional_context(self):
unconditional_tokens = tf.convert_to_tensor([UNCONDITIONAL_TOKENS], dtype="int32")
unconditional_context = self.text_encoder({"tokens": unconditional_tokens, "positions": self._get_pos_ids()})
return unconditional_context
def _expand_tensor(self, text_embedding, batch_size):
text_embedding = np.squeeze(text_embedding)
if len(text_embedding.shape) == 2:
text_embedding = np.repeat(np.expand_dims(text_embedding, axis=0), batch_size, axis=0)
return text_embedding
@property
def tokenizer(self):
if self._tokenizer is None:
self._tokenizer = SimpleTokenizer()
return self._tokenizer
def _call_ov_model(self, ov_model, args):
return ov_model(args)[ov_model.output(0)]
def _get_timestep_embedding(self, timestep, batch_size, dim=320, max_period=10000):
half = dim // 2
range = np.array(np.arange(0, half), "float32")
freqs = np.exp(-math.log(max_period) * range / half)
args = tf.convert_to_tensor([timestep], dtype="float32") * freqs
embedding = np.concatenate([np.cos(args), np.sin(args)], 0)
embedding = np.reshape(embedding, [1, -1])
return np.repeat(embedding, batch_size, axis=0)
def _get_initial_alphas(self, timesteps):
alphas = [ALPHAS_CUMPROD[t] for t in timesteps]
alphas_prev = [1.0] + alphas[:-1]
return alphas, alphas_prev
def _get_initial_diffusion_noise(self, batch_size, seed):
np.random.seed(seed)
return np.random.normal(
size=(batch_size, self.img_height // 8, self.img_width // 8, 4),
)
@staticmethod
def _get_pos_ids():
return np.expand_dims(np.arange(MAX_PROMPT_LENGTH, dtype="int32"), 0)
Select device from dropdown list for running inference using OpenVINO.
import ipywidgets as widgets
import openvino as ov
device = widgets.Dropdown(
options=ov.Core().available_devices + ["AUTO"],
value="AUTO",
description="Device:",
disabled=False,
)
device
Dropdown(description='Device:', index=4, options=('CPU', 'GPU.0', 'GPU.1', 'GPU.2', 'AUTO'), value='AUTO')
Read and compile pipeline models using selected device.
core = ov.Core()
ov_text_encoder = core.compile_model(OV_TEXT_ENCODER_MODEL_PATH, device.value)
ov_diffusion_model = core.compile_model(OV_DIFFUSION_MODEL_PATH, device.value)
ov_decoder = core.compile_model(OV_DECODER_MODEL_PATH, device.value)
import matplotlib.pyplot as plt
def plot_images(images):
plt.figure(figsize=(8 * len(images), 10))
for i in range(len(images)):
plt.subplot(1, len(images), i + 1)
plt.imshow(images[i])
plt.axis("off")
Create and run Stable Diffusion pipeline using OpenVINO models.
ov_pipeline = StableDiffusion(text_encoder=ov_text_encoder, diffusion_model=ov_diffusion_model, decoder=ov_decoder)
images = ov_pipeline.text_to_image("photograph of an astronaut riding a horse", num_steps=50, seed=80)
plot_images(images)
By using this model checkpoint, you acknowledge that its usage is subject to the terms of the CreativeML Open RAIL-M license at https://raw.githubusercontent.com/CompVis/stable-diffusion/main/LICENSE
50/50 [==============================] - 65s 1s/step
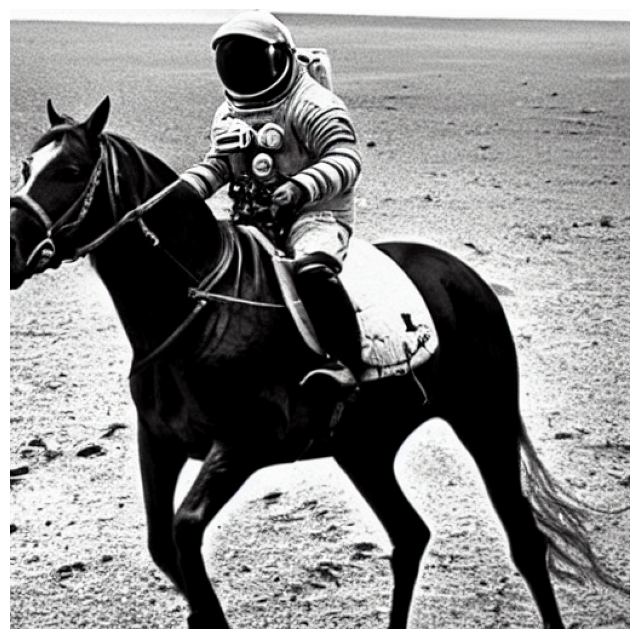
Quantization#
NNCF enables
post-training quantization by adding quantization layers into model
graph and then using a subset of the training dataset to initialize the
parameters of these additional quantization layers. Quantized operations
are executed in INT8
instead of FP32
/FP16
making model
inference faster.
According to keras_cv.models.StableDiffusion
structure, the
diffusion model takes up significant portion of the overall pipeline
execution time. Now we will show you how to optimize the UNet part using
NNCF to reduce
computation cost and speed up the pipeline. Quantizing the rest of the
pipeline does not significantly improve inference performance but can
lead to a substantial degradation of accuracy. That’s why we use weight
compression for text_encoder
and decoder
to reduce memory
footprint.
For the diffusion model we apply quantization in hybrid mode which means that we quantize: (1) weights of MatMul and Embedding layers and (2) activations of other layers. The steps are the following:
Create a calibration dataset for quantization.
Collect operations with weights.
Run
nncf.compress_model()
to compress only the model weights.Run
nncf.quantize()
on the compressed model with weighted operations ignored by providingignored_scope
parameter.Save the
INT8
model usingopenvino.save_model()
function.
Please select below whether you would like to run quantization to improve model inference speed.
NOTE: Quantization is time and memory consuming operation. Running quantization code below may take some time.
to_quantize = widgets.Checkbox(
value=True,
description="Quantization",
disabled=False,
)
to_quantize
Checkbox(value=True, description='Quantization')
# Fetch `skip_kernel_extension` module
import requests
r = requests.get(
url="https://raw.githubusercontent.com/openvinotoolkit/openvino_notebooks/latest/utils/skip_kernel_extension.py",
)
open("skip_kernel_extension.py", "w").write(r.text)
ov_int8_pipeline = None
OV_INT8_DIFFUSION_MODEL_PATH = Path("models/ov_int8_diffusion_model.xml")
OV_INT8_TEXT_ENCODER_MODEL_PATH = Path("models/ov_int8_text_encoder_model.xml")
OV_INT8_DECODER_MODEL_PATH = Path("models/ov_int8_decoder_model.xml")
%load_ext skip_kernel_extension
Prepare calibration dataset#
We use a portion of
conceptual_captions
dataset from Hugging Face as calibration data. To collect intermediate
model inputs for UNet optimization we should customize
CompiledModel
.
%%skip not $to_quantize.value
import datasets
import numpy as np
from tqdm.notebook import tqdm
from typing import Any, Dict, List
class CompiledModelDecorator(ov.CompiledModel):
def __init__(self, compiled_model: ov.CompiledModel, data_cache: List[Any] = None, keep_prob: float = 0.5):
super().__init__(compiled_model)
self.data_cache = data_cache if data_cache is not None else []
self.keep_prob = keep_prob
def __call__(self, *args, **kwargs):
if np.random.rand() <= self.keep_prob:
self.data_cache.append(*args)
return super().__call__(*args, **kwargs)
def collect_calibration_data(ov_pipe, calibration_dataset_size: int, num_inference_steps: int = 50) -> List[Dict]:
original_unet = ov_pipe._diffusion_model
calibration_data = []
ov_pipe._diffusion_model = CompiledModelDecorator(original_unet, calibration_data, keep_prob=0.7)
dataset = datasets.load_dataset("google-research-datasets/conceptual_captions", split="train", streaming=True, trust_remote_code=True).shuffle(seed=42)
# Run inference for data collection
pbar = tqdm(total=calibration_dataset_size)
for batch in dataset:
prompt = batch["caption"]
if len(prompt) > MAX_PROMPT_LENGTH:
continue
ov_pipe.text_to_image(prompt, num_steps=num_inference_steps, seed=1)
pbar.update(len(calibration_data) - pbar.n)
if pbar.n >= calibration_dataset_size:
break
ov_pipe._diffusion_model = original_unet
return calibration_data[:calibration_dataset_size]
%%skip not $to_quantize.value
if not OV_INT8_DIFFUSION_MODEL_PATH.exists() :
subset_size = 200
calibration_data = collect_calibration_data(ov_pipeline, calibration_dataset_size=subset_size)
/home/ltalamanova/tmp_venv/lib/python3.11/site-packages/datasets/load.py:1461: FutureWarning: The repository for conceptual_captions contains custom code which must be executed to correctly load the dataset. You can inspect the repository content at https://hf.co/datasets/conceptual_captions You can avoid this message in future by passing the argument trust_remote_code=True. Passing trust_remote_code=True will be mandatory to load this dataset from the next major release of datasets. warnings.warn(
0%| | 0/200 [00:00<?, ?it/s]
50/50 [==============================] - 65s 1s/step
50/50 [==============================] - 65s 1s/step
50/50 [==============================] - 65s 1s/step
Run Quantization#
%%skip not $to_quantize.value
from collections import deque
def get_operation_const_op(operation, const_port_id: int):
node = operation.input_value(const_port_id).get_node()
queue = deque([node])
constant_node = None
allowed_propagation_types_list = ["Convert", "FakeQuantize", "Reshape"]
while len(queue) != 0:
curr_node = queue.popleft()
if curr_node.get_type_name() == "Constant":
constant_node = curr_node
break
if len(curr_node.inputs()) == 0:
break
if curr_node.get_type_name() in allowed_propagation_types_list:
queue.append(curr_node.input_value(0).get_node())
return constant_node
def is_embedding(node) -> bool:
allowed_types_list = ["f16", "f32", "f64"]
const_port_id = 0
input_tensor = node.input_value(const_port_id)
if input_tensor.get_element_type().get_type_name() in allowed_types_list:
const_node = get_operation_const_op(node, const_port_id)
if const_node is not None:
return True
return False
def collect_ops_with_weights(model):
ops_with_weights = []
for op in model.get_ops():
if op.get_type_name() == "MatMul":
constant_node_0 = get_operation_const_op(op, const_port_id=0)
constant_node_1 = get_operation_const_op(op, const_port_id=1)
if constant_node_0 or constant_node_1:
ops_with_weights.append(op.get_friendly_name())
if op.get_type_name() == "Gather" and is_embedding(op):
ops_with_weights.append(op.get_friendly_name())
return ops_with_weights
%%skip not $to_quantize.value
import nncf
from nncf.quantization.advanced_parameters import AdvancedSmoothQuantParameters
if not OV_INT8_DIFFUSION_MODEL_PATH.exists():
diffusion_model = core.read_model(OV_DIFFUSION_MODEL_PATH)
unet_ignored_scope = collect_ops_with_weights(diffusion_model)
compressed_diffusion_model = nncf.compress_weights(diffusion_model, ignored_scope=nncf.IgnoredScope(types=['Convolution']))
quantized_diffusion_model = nncf.quantize(
model=compressed_diffusion_model,
calibration_dataset=nncf.Dataset(calibration_data),
subset_size=subset_size,
model_type=nncf.ModelType.TRANSFORMER,
ignored_scope=nncf.IgnoredScope(names=unet_ignored_scope),
advanced_parameters=nncf.AdvancedQuantizationParameters(smooth_quant_alphas=AdvancedSmoothQuantParameters(matmul=-1))
)
ov.save_model(quantized_diffusion_model, OV_INT8_DIFFUSION_MODEL_PATH)
INFO:nncf:NNCF initialized successfully. Supported frameworks detected: torch, tensorflow, onnx, openvino
INFO:nncf:98 ignored nodes were found by types in the NNCFGraph
INFO:nncf:Statistics of the bitwidth distribution:
+--------------+---------------------------+-----------------------------------+
| Num bits (N) | % all parameters (layers) | % ratio-defining parameters |
| | | (layers) |
+==============+===========================+===================================+
| 8 | 100% (184 / 184) | 100% (184 / 184) |
+--------------+---------------------------+-----------------------------------+
Output()
INFO:nncf:184 ignored nodes were found by name in the NNCFGraph
INFO:nncf:128 ignored nodes were found by name in the NNCFGraph
INFO:nncf:Not adding activation input quantizer for operation: 4 diffusion_model/dense_72/MatMul
8 diffusion_model/dense_72/BiasAdd
44 diffusion_model/activation/mul_1
INFO:nncf:Not adding activation input quantizer for operation: 10 diffusion_model/spatial_transformer/basic_transformer_block/cross_attention_1/dense_81/Tensordot/MatMul
INFO:nncf:Not adding activation input quantizer for operation: 11 diffusion_model/spatial_transformer_1/basic_transformer_block_1/cross_attention_3/dense_91/Tensordot/MatMul
INFO:nncf:Not adding activation input quantizer for operation: 12 diffusion_model/spatial_transformer_1/basic_transformer_block_1/cross_attention_3/dense_92/Tensordot/MatMul
INFO:nncf:Not adding activation input quantizer for operation: 13 diffusion_model/spatial_transformer_10/basic_transformer_block_10/cross_attention_21/dense_196/Tensordot/MatMul
INFO:nncf:Not adding activation input quantizer for operation: 14 diffusion_model/spatial_transformer_10/basic_transformer_block_10/cross_attention_21/dense_197/Tensordot/MatMul
INFO:nncf:Not adding activation input quantizer for operation: 15 diffusion_model/spatial_transformer_11/basic_transformer_block_11/cross_attention_23/dense_207/Tensordot/MatMul
INFO:nncf:Not adding activation input quantizer for operation: 16 diffusion_model/spatial_transformer_11/basic_transformer_block_11/cross_attention_23/dense_208/Tensordot/MatMul
INFO:nncf:Not adding activation input quantizer for operation: 17 diffusion_model/spatial_transformer_12/basic_transformer_block_12/cross_attention_25/dense_218/Tensordot/MatMul
INFO:nncf:Not adding activation input quantizer for operation: 18 diffusion_model/spatial_transformer_12/basic_transformer_block_12/cross_attention_25/dense_219/Tensordot/MatMul
INFO:nncf:Not adding activation input quantizer for operation: 19 diffusion_model/spatial_transformer_13/basic_transformer_block_13/cross_attention_27/dense_229/Tensordot/MatMul
INFO:nncf:Not adding activation input quantizer for operation: 20 diffusion_model/spatial_transformer_13/basic_transformer_block_13/cross_attention_27/dense_230/Tensordot/MatMul
INFO:nncf:Not adding activation input quantizer for operation: 21 diffusion_model/spatial_transformer_14/basic_transformer_block_14/cross_attention_29/dense_240/Tensordot/MatMul
INFO:nncf:Not adding activation input quantizer for operation: 22 diffusion_model/spatial_transformer_14/basic_transformer_block_14/cross_attention_29/dense_241/Tensordot/MatMul
INFO:nncf:Not adding activation input quantizer for operation: 23 diffusion_model/spatial_transformer_15/basic_transformer_block_15/cross_attention_31/dense_251/Tensordot/MatMul
INFO:nncf:Not adding activation input quantizer for operation: 24 diffusion_model/spatial_transformer_15/basic_transformer_block_15/cross_attention_31/dense_252/Tensordot/MatMul
INFO:nncf:Not adding activation input quantizer for operation: 25 diffusion_model/spatial_transformer_2/basic_transformer_block_2/cross_attention_5/dense_102/Tensordot/MatMul
INFO:nncf:Not adding activation input quantizer for operation: 26 diffusion_model/spatial_transformer_2/basic_transformer_block_2/cross_attention_5/dense_103/Tensordot/MatMul
INFO:nncf:Not adding activation input quantizer for operation: 27 diffusion_model/spatial_transformer_3/basic_transformer_block_3/cross_attention_7/dense_113/Tensordot/MatMul
INFO:nncf:Not adding activation input quantizer for operation: 28 diffusion_model/spatial_transformer_3/basic_transformer_block_3/cross_attention_7/dense_114/Tensordot/MatMul
INFO:nncf:Not adding activation input quantizer for operation: 29 diffusion_model/spatial_transformer_4/basic_transformer_block_4/cross_attention_9/dense_124/Tensordot/MatMul
INFO:nncf:Not adding activation input quantizer for operation: 30 diffusion_model/spatial_transformer_4/basic_transformer_block_4/cross_attention_9/dense_125/Tensordot/MatMul
INFO:nncf:Not adding activation input quantizer for operation: 31 diffusion_model/spatial_transformer_5/basic_transformer_block_5/cross_attention_11/dense_135/Tensordot/MatMul
INFO:nncf:Not adding activation input quantizer for operation: 32 diffusion_model/spatial_transformer_5/basic_transformer_block_5/cross_attention_11/dense_136/Tensordot/MatMul
INFO:nncf:Not adding activation input quantizer for operation: 33 diffusion_model/spatial_transformer_6/basic_transformer_block_6/cross_attention_13/dense_148/Tensordot/MatMul
INFO:nncf:Not adding activation input quantizer for operation: 34 diffusion_model/spatial_transformer_6/basic_transformer_block_6/cross_attention_13/dense_149/Tensordot/MatMul
INFO:nncf:Not adding activation input quantizer for operation: 35 diffusion_model/spatial_transformer_7/basic_transformer_block_7/cross_attention_15/dense_163/Tensordot/MatMul
INFO:nncf:Not adding activation input quantizer for operation: 36 diffusion_model/spatial_transformer_7/basic_transformer_block_7/cross_attention_15/dense_164/Tensordot/MatMul
INFO:nncf:Not adding activation input quantizer for operation: 37 diffusion_model/spatial_transformer_8/basic_transformer_block_8/cross_attention_17/dense_174/Tensordot/MatMul
INFO:nncf:Not adding activation input quantizer for operation: 38 diffusion_model/spatial_transformer_8/basic_transformer_block_8/cross_attention_17/dense_175/Tensordot/MatMul
INFO:nncf:Not adding activation input quantizer for operation: 39 diffusion_model/spatial_transformer_9/basic_transformer_block_9/cross_attention_19/dense_185/Tensordot/MatMul
INFO:nncf:Not adding activation input quantizer for operation: 40 diffusion_model/spatial_transformer_9/basic_transformer_block_9/cross_attention_19/dense_186/Tensordot/MatMul
INFO:nncf:Not adding activation input quantizer for operation: 84 diffusion_model/dense_73/MatMul
122 diffusion_model/dense_73/BiasAdd
168 diffusion_model/res_block/activation_2/mul_1
INFO:nncf:Not adding activation input quantizer for operation: 218 diffusion_model/res_block/dense_74/MatMul
287 diffusion_model/res_block/dense_74/BiasAdd
INFO:nncf:Not adding activation input quantizer for operation: 219 diffusion_model/res_block_1/dense_85/MatMul
288 diffusion_model/res_block_1/dense_85/BiasAdd
INFO:nncf:Not adding activation input quantizer for operation: 220 diffusion_model/res_block_10/dense_154/MatMul
289 diffusion_model/res_block_10/dense_154/BiasAdd
INFO:nncf:Not adding activation input quantizer for operation: 221 diffusion_model/res_block_11/dense_155/MatMul
290 diffusion_model/res_block_11/dense_155/BiasAdd
INFO:nncf:Not adding activation input quantizer for operation: 222 diffusion_model/res_block_12/dense_156/MatMul
291 diffusion_model/res_block_12/dense_156/BiasAdd
INFO:nncf:Not adding activation input quantizer for operation: 223 diffusion_model/res_block_13/dense_157/MatMul
292 diffusion_model/res_block_13/dense_157/BiasAdd
INFO:nncf:Not adding activation input quantizer for operation: 224 diffusion_model/res_block_14/dense_168/MatMul
293 diffusion_model/res_block_14/dense_168/BiasAdd
INFO:nncf:Not adding activation input quantizer for operation: 225 diffusion_model/res_block_15/dense_179/MatMul
294 diffusion_model/res_block_15/dense_179/BiasAdd
INFO:nncf:Not adding activation input quantizer for operation: 226 diffusion_model/res_block_16/dense_190/MatMul
295 diffusion_model/res_block_16/dense_190/BiasAdd
INFO:nncf:Not adding activation input quantizer for operation: 227 diffusion_model/res_block_17/dense_201/MatMul
296 diffusion_model/res_block_17/dense_201/BiasAdd
INFO:nncf:Not adding activation input quantizer for operation: 228 diffusion_model/res_block_18/dense_212/MatMul
297 diffusion_model/res_block_18/dense_212/BiasAdd
INFO:nncf:Not adding activation input quantizer for operation: 229 diffusion_model/res_block_19/dense_223/MatMul
298 diffusion_model/res_block_19/dense_223/BiasAdd
INFO:nncf:Not adding activation input quantizer for operation: 230 diffusion_model/res_block_2/dense_96/MatMul
299 diffusion_model/res_block_2/dense_96/BiasAdd
INFO:nncf:Not adding activation input quantizer for operation: 231 diffusion_model/res_block_20/dense_234/MatMul
300 diffusion_model/res_block_20/dense_234/BiasAdd
INFO:nncf:Not adding activation input quantizer for operation: 232 diffusion_model/res_block_21/dense_245/MatMul
301 diffusion_model/res_block_21/dense_245/BiasAdd
INFO:nncf:Not adding activation input quantizer for operation: 233 diffusion_model/res_block_3/dense_107/MatMul
302 diffusion_model/res_block_3/dense_107/BiasAdd
INFO:nncf:Not adding activation input quantizer for operation: 234 diffusion_model/res_block_4/dense_118/MatMul
303 diffusion_model/res_block_4/dense_118/BiasAdd
INFO:nncf:Not adding activation input quantizer for operation: 235 diffusion_model/res_block_5/dense_129/MatMul
304 diffusion_model/res_block_5/dense_129/BiasAdd
INFO:nncf:Not adding activation input quantizer for operation: 236 diffusion_model/res_block_6/dense_140/MatMul
305 diffusion_model/res_block_6/dense_140/BiasAdd
INFO:nncf:Not adding activation input quantizer for operation: 237 diffusion_model/res_block_7/dense_141/MatMul
306 diffusion_model/res_block_7/dense_141/BiasAdd
INFO:nncf:Not adding activation input quantizer for operation: 238 diffusion_model/res_block_8/dense_142/MatMul
307 diffusion_model/res_block_8/dense_142/BiasAdd
INFO:nncf:Not adding activation input quantizer for operation: 239 diffusion_model/res_block_9/dense_153/MatMul
308 diffusion_model/res_block_9/dense_153/BiasAdd
INFO:nncf:Not adding activation input quantizer for operation: 9 diffusion_model/spatial_transformer/basic_transformer_block/cross_attention_1/dense_80/Tensordot/MatMul
INFO:nncf:Not adding activation input quantizer for operation: 2355 diffusion_model/spatial_transformer/basic_transformer_block/cross_attention/dense_75/Tensordot/MatMul
INFO:nncf:Not adding activation input quantizer for operation: 2356 diffusion_model/spatial_transformer/basic_transformer_block/cross_attention/dense_76/Tensordot/MatMul
INFO:nncf:Not adding activation input quantizer for operation: 2357 diffusion_model/spatial_transformer/basic_transformer_block/cross_attention/dense_77/Tensordot/MatMul
INFO:nncf:Not adding activation input quantizer for operation: 5423 diffusion_model/spatial_transformer/basic_transformer_block/cross_attention/dense_78/Tensordot/MatMul
INFO:nncf:Not adding activation input quantizer for operation: 2691 diffusion_model/spatial_transformer/basic_transformer_block/cross_attention_1/dense_79/Tensordot/MatMul
INFO:nncf:Not adding activation input quantizer for operation: 709 diffusion_model/spatial_transformer/basic_transformer_block/cross_attention_1/dense_82/Tensordot/MatMul
INFO:nncf:Not adding activation input quantizer for operation: 2937 diffusion_model/spatial_transformer/basic_transformer_block/geglu/dense_83/Tensordot/MatMul
INFO:nncf:Not adding activation input quantizer for operation: 4990 diffusion_model/spatial_transformer/basic_transformer_block/dense_84/Tensordot/MatMul
INFO:nncf:Not adding activation input quantizer for operation: 4114 diffusion_model/spatial_transformer_1/basic_transformer_block_1/cross_attention_2/dense_86/Tensordot/MatMul
INFO:nncf:Not adding activation input quantizer for operation: 4115 diffusion_model/spatial_transformer_1/basic_transformer_block_1/cross_attention_2/dense_87/Tensordot/MatMul
INFO:nncf:Not adding activation input quantizer for operation: 4116 diffusion_model/spatial_transformer_1/basic_transformer_block_1/cross_attention_2/dense_88/Tensordot/MatMul
INFO:nncf:Not adding activation input quantizer for operation: 6228 diffusion_model/spatial_transformer_1/basic_transformer_block_1/cross_attention_2/dense_89/Tensordot/MatMul
INFO:nncf:Not adding activation input quantizer for operation: 4446 diffusion_model/spatial_transformer_1/basic_transformer_block_1/cross_attention_3/dense_90/Tensordot/MatMul
INFO:nncf:Not adding activation input quantizer for operation: 711 diffusion_model/spatial_transformer_1/basic_transformer_block_1/cross_attention_3/dense_93/Tensordot/MatMul
INFO:nncf:Not adding activation input quantizer for operation: 2940 diffusion_model/spatial_transformer_1/basic_transformer_block_1/geglu_1/dense_94/Tensordot/MatMul
INFO:nncf:Not adding activation input quantizer for operation: 4993 diffusion_model/spatial_transformer_1/basic_transformer_block_1/dense_95/Tensordot/MatMul
INFO:nncf:Not adding activation input quantizer for operation: 5955 diffusion_model/spatial_transformer_2/basic_transformer_block_2/cross_attention_4/dense_97/Tensordot/MatMul
INFO:nncf:Not adding activation input quantizer for operation: 5956 diffusion_model/spatial_transformer_2/basic_transformer_block_2/cross_attention_4/dense_98/Tensordot/MatMul
INFO:nncf:Not adding activation input quantizer for operation: 5957 diffusion_model/spatial_transformer_2/basic_transformer_block_2/cross_attention_4/dense_99/Tensordot/MatMul
INFO:nncf:Not adding activation input quantizer for operation: 6511 diffusion_model/spatial_transformer_2/basic_transformer_block_2/cross_attention_4/dense_100/Tensordot/MatMul
INFO:nncf:Not adding activation input quantizer for operation: 6091 diffusion_model/spatial_transformer_2/basic_transformer_block_2/cross_attention_5/dense_101/Tensordot/MatMul
INFO:nncf:Not adding activation input quantizer for operation: 725 diffusion_model/spatial_transformer_2/basic_transformer_block_2/cross_attention_5/dense_104/Tensordot/MatMul
INFO:nncf:Not adding activation input quantizer for operation: 2961 diffusion_model/spatial_transformer_2/basic_transformer_block_2/geglu_2/dense_105/Tensordot/MatMul
INFO:nncf:Not adding activation input quantizer for operation: 5023 diffusion_model/spatial_transformer_2/basic_transformer_block_2/dense_106/Tensordot/MatMul
INFO:nncf:Not adding activation input quantizer for operation: 5962 diffusion_model/spatial_transformer_3/basic_transformer_block_3/cross_attention_6/dense_108/Tensordot/MatMul
INFO:nncf:Not adding activation input quantizer for operation: 5963 diffusion_model/spatial_transformer_3/basic_transformer_block_3/cross_attention_6/dense_109/Tensordot/MatMul
INFO:nncf:Not adding activation input quantizer for operation: 5964 diffusion_model/spatial_transformer_3/basic_transformer_block_3/cross_attention_6/dense_110/Tensordot/MatMul
INFO:nncf:Not adding activation input quantizer for operation: 6513 diffusion_model/spatial_transformer_3/basic_transformer_block_3/cross_attention_6/dense_111/Tensordot/MatMul
INFO:nncf:Not adding activation input quantizer for operation: 6099 diffusion_model/spatial_transformer_3/basic_transformer_block_3/cross_attention_7/dense_112/Tensordot/MatMul
INFO:nncf:Not adding activation input quantizer for operation: 727 diffusion_model/spatial_transformer_3/basic_transformer_block_3/cross_attention_7/dense_115/Tensordot/MatMul
INFO:nncf:Not adding activation input quantizer for operation: 2964 diffusion_model/spatial_transformer_3/basic_transformer_block_3/geglu_3/dense_116/Tensordot/MatMul
INFO:nncf:Not adding activation input quantizer for operation: 5034 diffusion_model/spatial_transformer_3/basic_transformer_block_3/dense_117/Tensordot/MatMul
INFO:nncf:Not adding activation input quantizer for operation: 5969 diffusion_model/spatial_transformer_4/basic_transformer_block_4/cross_attention_8/dense_119/Tensordot/MatMul
INFO:nncf:Not adding activation input quantizer for operation: 5970 diffusion_model/spatial_transformer_4/basic_transformer_block_4/cross_attention_8/dense_120/Tensordot/MatMul
INFO:nncf:Not adding activation input quantizer for operation: 5971 diffusion_model/spatial_transformer_4/basic_transformer_block_4/cross_attention_8/dense_121/Tensordot/MatMul
INFO:nncf:Not adding activation input quantizer for operation: 6515 diffusion_model/spatial_transformer_4/basic_transformer_block_4/cross_attention_8/dense_122/Tensordot/MatMul
INFO:nncf:Not adding activation input quantizer for operation: 6107 diffusion_model/spatial_transformer_4/basic_transformer_block_4/cross_attention_9/dense_123/Tensordot/MatMul
INFO:nncf:Not adding activation input quantizer for operation: 729 diffusion_model/spatial_transformer_4/basic_transformer_block_4/cross_attention_9/dense_126/Tensordot/MatMul
INFO:nncf:Not adding activation input quantizer for operation: 2967 diffusion_model/spatial_transformer_4/basic_transformer_block_4/geglu_4/dense_127/Tensordot/MatMul
INFO:nncf:Not adding activation input quantizer for operation: 5058 diffusion_model/spatial_transformer_4/basic_transformer_block_4/dense_128/Tensordot/MatMul
INFO:nncf:Not adding activation input quantizer for operation: 5976 diffusion_model/spatial_transformer_5/basic_transformer_block_5/cross_attention_10/dense_130/Tensordot/MatMul
INFO:nncf:Not adding activation input quantizer for operation: 5977 diffusion_model/spatial_transformer_5/basic_transformer_block_5/cross_attention_10/dense_131/Tensordot/MatMul
INFO:nncf:Not adding activation input quantizer for operation: 5978 diffusion_model/spatial_transformer_5/basic_transformer_block_5/cross_attention_10/dense_132/Tensordot/MatMul
INFO:nncf:Not adding activation input quantizer for operation: 6517 diffusion_model/spatial_transformer_5/basic_transformer_block_5/cross_attention_10/dense_133/Tensordot/MatMul
INFO:nncf:Not adding activation input quantizer for operation: 6115 diffusion_model/spatial_transformer_5/basic_transformer_block_5/cross_attention_11/dense_134/Tensordot/MatMul
INFO:nncf:Not adding activation input quantizer for operation: 731 diffusion_model/spatial_transformer_5/basic_transformer_block_5/cross_attention_11/dense_137/Tensordot/MatMul
INFO:nncf:Not adding activation input quantizer for operation: 2970 diffusion_model/spatial_transformer_5/basic_transformer_block_5/geglu_5/dense_138/Tensordot/MatMul
INFO:nncf:Not adding activation input quantizer for operation: 5069 diffusion_model/spatial_transformer_5/basic_transformer_block_5/dense_139/Tensordot/MatMul
INFO:nncf:Not adding activation input quantizer for operation: 5983 diffusion_model/spatial_transformer_6/basic_transformer_block_6/cross_attention_12/dense_143/Tensordot/MatMul
INFO:nncf:Not adding activation input quantizer for operation: 5984 diffusion_model/spatial_transformer_6/basic_transformer_block_6/cross_attention_12/dense_144/Tensordot/MatMul
INFO:nncf:Not adding activation input quantizer for operation: 5985 diffusion_model/spatial_transformer_6/basic_transformer_block_6/cross_attention_12/dense_145/Tensordot/MatMul
INFO:nncf:Not adding activation input quantizer for operation: 6519 diffusion_model/spatial_transformer_6/basic_transformer_block_6/cross_attention_12/dense_146/Tensordot/MatMul
INFO:nncf:Not adding activation input quantizer for operation: 6123 diffusion_model/spatial_transformer_6/basic_transformer_block_6/cross_attention_13/dense_147/Tensordot/MatMul
INFO:nncf:Not adding activation input quantizer for operation: 733 diffusion_model/spatial_transformer_6/basic_transformer_block_6/cross_attention_13/dense_150/Tensordot/MatMul
INFO:nncf:Not adding activation input quantizer for operation: 2973 diffusion_model/spatial_transformer_6/basic_transformer_block_6/geglu_6/dense_151/Tensordot/MatMul
INFO:nncf:Not adding activation input quantizer for operation: 5093 diffusion_model/spatial_transformer_6/basic_transformer_block_6/dense_152/Tensordot/MatMul
INFO:nncf:Not adding activation input quantizer for operation: 5913 diffusion_model/spatial_transformer_7/basic_transformer_block_7/cross_attention_14/dense_158/Tensordot/MatMul
INFO:nncf:Not adding activation input quantizer for operation: 5914 diffusion_model/spatial_transformer_7/basic_transformer_block_7/cross_attention_14/dense_159/Tensordot/MatMul
INFO:nncf:Not adding activation input quantizer for operation: 5915 diffusion_model/spatial_transformer_7/basic_transformer_block_7/cross_attention_14/dense_160/Tensordot/MatMul
INFO:nncf:Not adding activation input quantizer for operation: 6499 diffusion_model/spatial_transformer_7/basic_transformer_block_7/cross_attention_14/dense_161/Tensordot/MatMul
INFO:nncf:Not adding activation input quantizer for operation: 6043 diffusion_model/spatial_transformer_7/basic_transformer_block_7/cross_attention_15/dense_162/Tensordot/MatMul
INFO:nncf:Not adding activation input quantizer for operation: 735 diffusion_model/spatial_transformer_7/basic_transformer_block_7/cross_attention_15/dense_165/Tensordot/MatMul
INFO:nncf:Not adding activation input quantizer for operation: 2976 diffusion_model/spatial_transformer_7/basic_transformer_block_7/geglu_7/dense_166/Tensordot/MatMul
INFO:nncf:Not adding activation input quantizer for operation: 5104 diffusion_model/spatial_transformer_7/basic_transformer_block_7/dense_167/Tensordot/MatMul
INFO:nncf:Not adding activation input quantizer for operation: 5920 diffusion_model/spatial_transformer_8/basic_transformer_block_8/cross_attention_16/dense_169/Tensordot/MatMul
INFO:nncf:Not adding activation input quantizer for operation: 5921 diffusion_model/spatial_transformer_8/basic_transformer_block_8/cross_attention_16/dense_170/Tensordot/MatMul
INFO:nncf:Not adding activation input quantizer for operation: 5922 diffusion_model/spatial_transformer_8/basic_transformer_block_8/cross_attention_16/dense_171/Tensordot/MatMul
INFO:nncf:Not adding activation input quantizer for operation: 6501 diffusion_model/spatial_transformer_8/basic_transformer_block_8/cross_attention_16/dense_172/Tensordot/MatMul
INFO:nncf:Not adding activation input quantizer for operation: 6051 diffusion_model/spatial_transformer_8/basic_transformer_block_8/cross_attention_17/dense_173/Tensordot/MatMul
INFO:nncf:Not adding activation input quantizer for operation: 737 diffusion_model/spatial_transformer_8/basic_transformer_block_8/cross_attention_17/dense_176/Tensordot/MatMul
INFO:nncf:Not adding activation input quantizer for operation: 2979 diffusion_model/spatial_transformer_8/basic_transformer_block_8/geglu_8/dense_177/Tensordot/MatMul
INFO:nncf:Not adding activation input quantizer for operation: 5116 diffusion_model/spatial_transformer_8/basic_transformer_block_8/dense_178/Tensordot/MatMul
INFO:nncf:Not adding activation input quantizer for operation: 5927 diffusion_model/spatial_transformer_9/basic_transformer_block_9/cross_attention_18/dense_180/Tensordot/MatMul
INFO:nncf:Not adding activation input quantizer for operation: 5928 diffusion_model/spatial_transformer_9/basic_transformer_block_9/cross_attention_18/dense_181/Tensordot/MatMul
INFO:nncf:Not adding activation input quantizer for operation: 5929 diffusion_model/spatial_transformer_9/basic_transformer_block_9/cross_attention_18/dense_182/Tensordot/MatMul
INFO:nncf:Not adding activation input quantizer for operation: 6503 diffusion_model/spatial_transformer_9/basic_transformer_block_9/cross_attention_18/dense_183/Tensordot/MatMul
INFO:nncf:Not adding activation input quantizer for operation: 6059 diffusion_model/spatial_transformer_9/basic_transformer_block_9/cross_attention_19/dense_184/Tensordot/MatMul
INFO:nncf:Not adding activation input quantizer for operation: 739 diffusion_model/spatial_transformer_9/basic_transformer_block_9/cross_attention_19/dense_187/Tensordot/MatMul
INFO:nncf:Not adding activation input quantizer for operation: 2982 diffusion_model/spatial_transformer_9/basic_transformer_block_9/geglu_9/dense_188/Tensordot/MatMul
INFO:nncf:Not adding activation input quantizer for operation: 5128 diffusion_model/spatial_transformer_9/basic_transformer_block_9/dense_189/Tensordot/MatMul
INFO:nncf:Not adding activation input quantizer for operation: 5934 diffusion_model/spatial_transformer_10/basic_transformer_block_10/cross_attention_20/dense_191/Tensordot/MatMul
INFO:nncf:Not adding activation input quantizer for operation: 5935 diffusion_model/spatial_transformer_10/basic_transformer_block_10/cross_attention_20/dense_192/Tensordot/MatMul
INFO:nncf:Not adding activation input quantizer for operation: 5936 diffusion_model/spatial_transformer_10/basic_transformer_block_10/cross_attention_20/dense_193/Tensordot/MatMul
INFO:nncf:Not adding activation input quantizer for operation: 6505 diffusion_model/spatial_transformer_10/basic_transformer_block_10/cross_attention_20/dense_194/Tensordot/MatMul
INFO:nncf:Not adding activation input quantizer for operation: 6067 diffusion_model/spatial_transformer_10/basic_transformer_block_10/cross_attention_21/dense_195/Tensordot/MatMul
INFO:nncf:Not adding activation input quantizer for operation: 713 diffusion_model/spatial_transformer_10/basic_transformer_block_10/cross_attention_21/dense_198/Tensordot/MatMul
INFO:nncf:Not adding activation input quantizer for operation: 2943 diffusion_model/spatial_transformer_10/basic_transformer_block_10/geglu_10/dense_199/Tensordot/MatMul
INFO:nncf:Not adding activation input quantizer for operation: 4996 diffusion_model/spatial_transformer_10/basic_transformer_block_10/dense_200/Tensordot/MatMul
INFO:nncf:Not adding activation input quantizer for operation: 5941 diffusion_model/spatial_transformer_11/basic_transformer_block_11/cross_attention_22/dense_202/Tensordot/MatMul
INFO:nncf:Not adding activation input quantizer for operation: 5942 diffusion_model/spatial_transformer_11/basic_transformer_block_11/cross_attention_22/dense_203/Tensordot/MatMul
INFO:nncf:Not adding activation input quantizer for operation: 5943 diffusion_model/spatial_transformer_11/basic_transformer_block_11/cross_attention_22/dense_204/Tensordot/MatMul
INFO:nncf:Not adding activation input quantizer for operation: 6507 diffusion_model/spatial_transformer_11/basic_transformer_block_11/cross_attention_22/dense_205/Tensordot/MatMul
INFO:nncf:Not adding activation input quantizer for operation: 6075 diffusion_model/spatial_transformer_11/basic_transformer_block_11/cross_attention_23/dense_206/Tensordot/MatMul
INFO:nncf:Not adding activation input quantizer for operation: 715 diffusion_model/spatial_transformer_11/basic_transformer_block_11/cross_attention_23/dense_209/Tensordot/MatMul
INFO:nncf:Not adding activation input quantizer for operation: 2946 diffusion_model/spatial_transformer_11/basic_transformer_block_11/geglu_11/dense_210/Tensordot/MatMul
INFO:nncf:Not adding activation input quantizer for operation: 5008 diffusion_model/spatial_transformer_11/basic_transformer_block_11/dense_211/Tensordot/MatMul
INFO:nncf:Not adding activation input quantizer for operation: 5948 diffusion_model/spatial_transformer_12/basic_transformer_block_12/cross_attention_24/dense_213/Tensordot/MatMul
INFO:nncf:Not adding activation input quantizer for operation: 5949 diffusion_model/spatial_transformer_12/basic_transformer_block_12/cross_attention_24/dense_214/Tensordot/MatMul
INFO:nncf:Not adding activation input quantizer for operation: 5950 diffusion_model/spatial_transformer_12/basic_transformer_block_12/cross_attention_24/dense_215/Tensordot/MatMul
INFO:nncf:Not adding activation input quantizer for operation: 6509 diffusion_model/spatial_transformer_12/basic_transformer_block_12/cross_attention_24/dense_216/Tensordot/MatMul
INFO:nncf:Not adding activation input quantizer for operation: 6083 diffusion_model/spatial_transformer_12/basic_transformer_block_12/cross_attention_25/dense_217/Tensordot/MatMul
INFO:nncf:Not adding activation input quantizer for operation: 717 diffusion_model/spatial_transformer_12/basic_transformer_block_12/cross_attention_25/dense_220/Tensordot/MatMul
INFO:nncf:Not adding activation input quantizer for operation: 2949 diffusion_model/spatial_transformer_12/basic_transformer_block_12/geglu_12/dense_221/Tensordot/MatMul
INFO:nncf:Not adding activation input quantizer for operation: 5011 diffusion_model/spatial_transformer_12/basic_transformer_block_12/dense_222/Tensordot/MatMul
INFO:nncf:Not adding activation input quantizer for operation: 5900 diffusion_model/spatial_transformer_13/basic_transformer_block_13/cross_attention_26/dense_224/Tensordot/MatMul
INFO:nncf:Not adding activation input quantizer for operation: 5901 diffusion_model/spatial_transformer_13/basic_transformer_block_13/cross_attention_26/dense_225/Tensordot/MatMul
INFO:nncf:Not adding activation input quantizer for operation: 5902 diffusion_model/spatial_transformer_13/basic_transformer_block_13/cross_attention_26/dense_226/Tensordot/MatMul
INFO:nncf:Not adding activation input quantizer for operation: 6497 diffusion_model/spatial_transformer_13/basic_transformer_block_13/cross_attention_26/dense_227/Tensordot/MatMul
INFO:nncf:Not adding activation input quantizer for operation: 6030 diffusion_model/spatial_transformer_13/basic_transformer_block_13/cross_attention_27/dense_228/Tensordot/MatMul
INFO:nncf:Not adding activation input quantizer for operation: 719 diffusion_model/spatial_transformer_13/basic_transformer_block_13/cross_attention_27/dense_231/Tensordot/MatMul
INFO:nncf:Not adding activation input quantizer for operation: 2952 diffusion_model/spatial_transformer_13/basic_transformer_block_13/geglu_13/dense_232/Tensordot/MatMul
INFO:nncf:Not adding activation input quantizer for operation: 5014 diffusion_model/spatial_transformer_13/basic_transformer_block_13/dense_233/Tensordot/MatMul
INFO:nncf:Not adding activation input quantizer for operation: 5157 diffusion_model/spatial_transformer_14/basic_transformer_block_14/cross_attention_28/dense_235/Tensordot/MatMul
INFO:nncf:Not adding activation input quantizer for operation: 5158 diffusion_model/spatial_transformer_14/basic_transformer_block_14/cross_attention_28/dense_236/Tensordot/MatMul
INFO:nncf:Not adding activation input quantizer for operation: 5159 diffusion_model/spatial_transformer_14/basic_transformer_block_14/cross_attention_28/dense_237/Tensordot/MatMul
INFO:nncf:Not adding activation input quantizer for operation: 6386 diffusion_model/spatial_transformer_14/basic_transformer_block_14/cross_attention_28/dense_238/Tensordot/MatMul
INFO:nncf:Not adding activation input quantizer for operation: 5447 diffusion_model/spatial_transformer_14/basic_transformer_block_14/cross_attention_29/dense_239/Tensordot/MatMul
INFO:nncf:Not adding activation input quantizer for operation: 721 diffusion_model/spatial_transformer_14/basic_transformer_block_14/cross_attention_29/dense_242/Tensordot/MatMul
INFO:nncf:Not adding activation input quantizer for operation: 2955 diffusion_model/spatial_transformer_14/basic_transformer_block_14/geglu_14/dense_243/Tensordot/MatMul
INFO:nncf:Not adding activation input quantizer for operation: 5017 diffusion_model/spatial_transformer_14/basic_transformer_block_14/dense_244/Tensordot/MatMul
INFO:nncf:Not adding activation input quantizer for operation: 3266 diffusion_model/spatial_transformer_15/basic_transformer_block_15/cross_attention_30/dense_246/Tensordot/MatMul
INFO:nncf:Not adding activation input quantizer for operation: 3267 diffusion_model/spatial_transformer_15/basic_transformer_block_15/cross_attention_30/dense_247/Tensordot/MatMul
INFO:nncf:Not adding activation input quantizer for operation: 3268 diffusion_model/spatial_transformer_15/basic_transformer_block_15/cross_attention_30/dense_248/Tensordot/MatMul
INFO:nncf:Not adding activation input quantizer for operation: 5911 diffusion_model/spatial_transformer_15/basic_transformer_block_15/cross_attention_30/dense_249/Tensordot/MatMul
INFO:nncf:Not adding activation input quantizer for operation: 3531 diffusion_model/spatial_transformer_15/basic_transformer_block_15/cross_attention_31/dense_250/Tensordot/MatMul
INFO:nncf:Not adding activation input quantizer for operation: 723 diffusion_model/spatial_transformer_15/basic_transformer_block_15/cross_attention_31/dense_253/Tensordot/MatMul
INFO:nncf:Not adding activation input quantizer for operation: 2958 diffusion_model/spatial_transformer_15/basic_transformer_block_15/geglu_15/dense_254/Tensordot/MatMul
INFO:nncf:Not adding activation input quantizer for operation: 5020 diffusion_model/spatial_transformer_15/basic_transformer_block_15/dense_255/Tensordot/MatMul
Output()
Output()
Run Weight Compression#
Quantizing of the text encoder
and decoder
does not
significantly improve inference performance but can lead to a
substantial degradation of accuracy. The weight compression will be
applied to footprint reduction.
%%skip not $to_quantize.value
if not OV_INT8_TEXT_ENCODER_MODEL_PATH.exists():
text_encoder_model = core.read_model(OV_TEXT_ENCODER_MODEL_PATH)
compressed_text_encoder_model = nncf.compress_weights(text_encoder_model)
ov.save_model(compressed_text_encoder_model, OV_INT8_TEXT_ENCODER_MODEL_PATH)
if not OV_INT8_DECODER_MODEL_PATH.exists():
decoder_model = core.read_model(OV_DECODER_MODEL_PATH)
compressed_decoder_model = nncf.compress_weights(decoder_model)
ov.save_model(compressed_decoder_model, OV_INT8_DECODER_MODEL_PATH)
INFO:nncf:Statistics of the bitwidth distribution:
+--------------+---------------------------+-----------------------------------+
| Num bits (N) | % all parameters (layers) | % ratio-defining parameters |
| | | (layers) |
+==============+===========================+===================================+
| 8 | 100% (74 / 74) | 100% (74 / 74) |
+--------------+---------------------------+-----------------------------------+
Output()
INFO:nncf:Statistics of the bitwidth distribution:
+--------------+---------------------------+-----------------------------------+
| Num bits (N) | % all parameters (layers) | % ratio-defining parameters |
| | | (layers) |
+==============+===========================+===================================+
| 8 | 100% (40 / 40) | 100% (40 / 40) |
+--------------+---------------------------+-----------------------------------+
Output()
Let’s compare the images generated by the original and optimized pipelines.
%%skip not $to_quantize.value
ov_int8_text_encoder = core.compile_model(OV_INT8_TEXT_ENCODER_MODEL_PATH, device.value)
ov_int8_diffusion_model = core.compile_model(OV_INT8_DIFFUSION_MODEL_PATH, device.value)
ov_int8_decoder = core.compile_model(OV_INT8_DECODER_MODEL_PATH, device.value)
ov_int8_pipeline = StableDiffusion(
text_encoder=ov_int8_text_encoder, diffusion_model=ov_int8_diffusion_model, decoder=ov_int8_decoder,
)
int8_image = ov_int8_pipeline.text_to_image(
"photograph of an astronaut riding a horse",
num_steps=50,
seed=80
)[0]
By using this model checkpoint, you acknowledge that its usage is subject to the terms of the CreativeML Open RAIL-M license at https://raw.githubusercontent.com/CompVis/stable-diffusion/main/LICENSE
50/50 [==============================] - 39s 785ms/step
%%skip not $to_quantize.value
import matplotlib.pyplot as plt
def visualize_results(orig_img, optimized_img):
"""
Helper function for results visualization
Parameters:
orig_img (Image.Image): generated image using FP16 models
optimized_img (Image.Image): generated image using quantized models
Returns:
fig (matplotlib.pyplot.Figure): matplotlib generated figure contains drawing result
"""
orig_title = "FP16 pipeline"
control_title = "INT8 pipeline"
figsize = (20, 20)
fig, axs = plt.subplots(1, 2, figsize=figsize, sharex='all', sharey='all')
list_axes = list(axs.flat)
for a in list_axes:
a.set_xticklabels([])
a.set_yticklabels([])
a.get_xaxis().set_visible(False)
a.get_yaxis().set_visible(False)
a.grid(False)
list_axes[0].imshow(np.array(orig_img))
list_axes[1].imshow(np.array(optimized_img))
list_axes[0].set_title(orig_title, fontsize=15)
list_axes[1].set_title(control_title, fontsize=15)
fig.subplots_adjust(wspace=0.01, hspace=0.01)
fig.tight_layout()
return fig
%%skip not $to_quantize.value
visualize_results(images[0], int8_image)
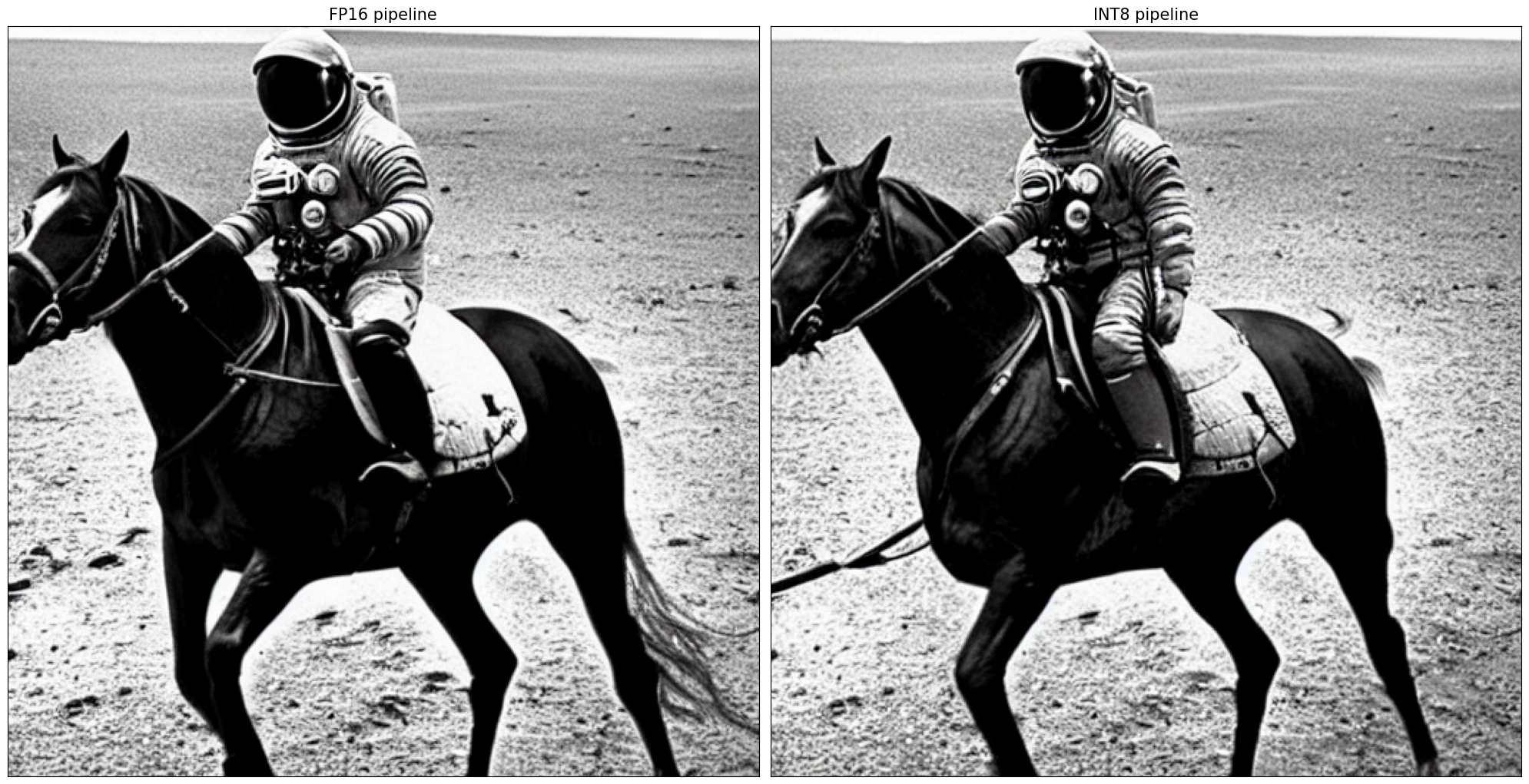
Compare model file sizes#
%%skip not $to_quantize.value
fp16_model_paths = [OV_TEXT_ENCODER_MODEL_PATH, OV_DIFFUSION_MODEL_PATH, OV_DECODER_MODEL_PATH]
int8_model_paths = [OV_INT8_TEXT_ENCODER_MODEL_PATH, OV_INT8_DIFFUSION_MODEL_PATH, OV_INT8_DECODER_MODEL_PATH]
for fp16_path, int8_path in zip(fp16_model_paths, int8_model_paths):
fp16_ir_model_size = fp16_path.with_suffix(".bin").stat().st_size
int8_model_size = int8_path.with_suffix(".bin").stat().st_size
print(f"{fp16_path.stem} compression rate: {fp16_ir_model_size / int8_model_size:.3f}")
ov_text_encoder_model compression rate: 1.992
ov_diffusion_model compression rate: 1.997
ov_decoder_model compression rate: 1.997
Compare inference time of the FP16 and INT8 pipelines#
To measure the inference performance of the FP16
and INT8
pipelines, we use median inference time on calibration subset.
NOTE: For the most accurate performance estimation, it is recommended to run
benchmark_app
in a terminal/command prompt after closing other applications.
%%skip not $to_quantize.value
import time
def calculate_inference_time(pipeline, validation_data):
inference_time = []
for prompt in validation_data:
start = time.perf_counter()
_ = pipeline.text_to_image(prompt, num_steps=50, seed=1)
end = time.perf_counter()
delta = end - start
inference_time.append(delta)
return np.median(inference_time)
%%skip not $to_quantize.value
validation_size = 3
validation_dataset = datasets.load_dataset("google-research-datasets/conceptual_captions", split="train", streaming=True, trust_remote_code=True).take(validation_size)
validation_data = [batch["caption"] for batch in validation_dataset]
fp_latency = calculate_inference_time(ov_pipeline, validation_data)
int8_latency = calculate_inference_time(ov_int8_pipeline, validation_data)
print(f"Performance speed-up: {fp_latency / int8_latency:.3f}")
/home/ltalamanova/tmp_venv/lib/python3.11/site-packages/datasets/load.py:1461: FutureWarning: The repository for conceptual_captions contains custom code which must be executed to correctly load the dataset. You can inspect the repository content at https://hf.co/datasets/conceptual_captions You can avoid this message in future by passing the argument trust_remote_code=True. Passing trust_remote_code=True will be mandatory to load this dataset from the next major release of datasets. warnings.warn(
50/50 [==============================] - 65s 1s/step
50/50 [==============================] - 65s 1s/step
50/50 [==============================] - 65s 1s/step
50/50 [==============================] - 39s 785ms/step
50/50 [==============================] - 39s 783ms/step
50/50 [==============================] - 39s 784ms/step
Performance speed-up: 1.628
Interactive Demo#
Please select below whether you would like to use the quantized model to launch the interactive demo.
use_quantized_model = widgets.Checkbox(
description="Use quantized model",
value=ov_int8_pipeline is not None,
disabled=ov_int8_pipeline is None,
)
use_quantized_model
Checkbox(value=True, description='Use quantized model')
import gradio as gr
pipeline = ov_int8_pipeline if use_quantized_model.value else ov_pipeline
def generate(text, seed, steps):
return pipeline.text_to_image(text, num_steps=steps, seed=seed)[0]
demo = gr.Interface(
generate,
[
gr.Textbox(lines=3, label="Text"),
gr.Slider(0, 10000000, value=45, label="Seed"),
gr.Slider(1, 50, value=25, step=1, label="Steps"),
],
gr.Image(label="Result"),
examples=[
["photograph of an astronaut riding a horse", 80, 25],
["photograph of a cat", 45, 25],
],
allow_flagging="never",
)
try:
demo.launch(debug=True, height=1000)
except Exception:
demo.launch(share=True, debug=True, height=1000)
# if you are launching remotely, specify server_name and server_port
# demo.launch(server_name='your server name', server_port='server port in int')
# Read more in the docs: https://gradio.app/docs/