Depth estimation with DepthAnything and OpenVINO¶
This Jupyter notebook can be launched on-line, opening an interactive environment in a browser window. You can also make a local installation. Choose one of the following options:
Depth Anything is a highly
practical solution for robust monocular depth estimation. Without
pursuing novel technical modules, this project aims to build a simple
yet powerful foundation model dealing with any images under any
circumstances. The framework of Depth Anything is shown below. it adopts
a standard pipeline to unleashing the power of large-scale unlabeled
images.
More details about model can be found in project web page, paper and official repository
In this tutorial we will explore how to convert and run DepthAnything using OpenVINO.
Table of contents:¶
Prerequisites¶
from pathlib import Path
repo_dir = Path("Depth-Anything")
if not repo_dir.exists():
!git clone https://github.com/LiheYoung/Depth-Anything
%cd Depth-Anything
%pip install -q "openvino>=2023.3.0"
%pip install -q "typing-extensions>=4.9.0" eval-type-backport
%pip install -q "gradio-imageslider<0.0.18"; python_version <= "3.9"
%pip install -q -r requirements.txt --extra-index-url https://download.pytorch.org/whl/cpu
Cloning into 'Depth-Anything'...
remote: Enumerating objects: 406, done.[K
remote: Counting objects: 0% (1/141)[K
remote: Counting objects: 1% (2/141)[K remote: Counting objects: 2% (3/141)[K remote: Counting objects: 3% (5/141)[K remote: Counting objects: 4% (6/141)[K remote: Counting objects: 5% (8/141)[K remote: Counting objects: 6% (9/141)[K remote: Counting objects: 7% (10/141)[K remote: Counting objects: 8% (12/141)[K remote: Counting objects: 9% (13/141)[K remote: Counting objects: 10% (15/141)[K remote: Counting objects: 11% (16/141)[K remote: Counting objects: 12% (17/141)[K remote: Counting objects: 13% (19/141)[K remote: Counting objects: 14% (20/141)[K remote: Counting objects: 15% (22/141)[K remote: Counting objects: 16% (23/141)[K remote: Counting objects: 17% (24/141)[K remote: Counting objects: 18% (26/141)[K remote: Counting objects: 19% (27/141)[K remote: Counting objects: 20% (29/141)[K remote: Counting objects: 21% (30/141)[K remote: Counting objects: 22% (32/141)[K remote: Counting objects: 23% (33/141)[K remote: Counting objects: 24% (34/141)[K remote: Counting objects: 25% (36/141)[K remote: Counting objects: 26% (37/141)[K remote: Counting objects: 27% (39/141)[K remote: Counting objects: 28% (40/141)[K remote: Counting objects: 29% (41/141)[K remote: Counting objects: 30% (43/141)[K remote: Counting objects: 31% (44/141)[K remote: Counting objects: 32% (46/141)[K remote: Counting objects: 33% (47/141)[K remote: Counting objects: 34% (48/141)[K remote: Counting objects: 35% (50/141)[K remote: Counting objects: 36% (51/141)[K remote: Counting objects: 37% (53/141)[K remote: Counting objects: 38% (54/141)[K remote: Counting objects: 39% (55/141)[K remote: Counting objects: 40% (57/141)[K remote: Counting objects: 41% (58/141)[K remote: Counting objects: 42% (60/141)[K remote: Counting objects: 43% (61/141)[K remote: Counting objects: 44% (63/141)[K remote: Counting objects: 45% (64/141)[K remote: Counting objects: 46% (65/141)[K remote: Counting objects: 47% (67/141)[K remote: Counting objects: 48% (68/141)[K remote: Counting objects: 49% (70/141)[K remote: Counting objects: 50% (71/141)[K remote: Counting objects: 51% (72/141)[K remote: Counting objects: 52% (74/141)[K remote: Counting objects: 53% (75/141)[K remote: Counting objects: 54% (77/141)[K remote: Counting objects: 55% (78/141)[K remote: Counting objects: 56% (79/141)[K remote: Counting objects: 57% (81/141)[K remote: Counting objects: 58% (82/141)[K remote: Counting objects: 59% (84/141)[K remote: Counting objects: 60% (85/141)[K remote: Counting objects: 61% (87/141)[K remote: Counting objects: 62% (88/141)[K remote: Counting objects: 63% (89/141)[K remote: Counting objects: 64% (91/141)[K remote: Counting objects: 65% (92/141)[K remote: Counting objects: 66% (94/141)[K remote: Counting objects: 67% (95/141)[K remote: Counting objects: 68% (96/141)[K remote: Counting objects: 69% (98/141)[K remote: Counting objects: 70% (99/141)[K remote: Counting objects: 71% (101/141)[K remote: Counting objects: 72% (102/141)[K remote: Counting objects: 73% (103/141)[K remote: Counting objects: 74% (105/141)[K remote: Counting objects: 75% (106/141)[K remote: Counting objects: 76% (108/141)[K remote: Counting objects: 77% (109/141)[K remote: Counting objects: 78% (110/141)[K remote: Counting objects: 79% (112/141)[K remote: Counting objects: 80% (113/141)[K remote: Counting objects: 81% (115/141)[K remote: Counting objects: 82% (116/141)[K remote: Counting objects: 83% (118/141)[K remote: Counting objects: 84% (119/141)[K remote: Counting objects: 85% (120/141)[K remote: Counting objects: 86% (122/141)[K remote: Counting objects: 87% (123/141)[K remote: Counting objects: 88% (125/141)[K remote: Counting objects: 89% (126/141)[K remote: Counting objects: 90% (127/141)[K remote: Counting objects: 91% (129/141)[K remote: Counting objects: 92% (130/141)[K remote: Counting objects: 93% (132/141)[K remote: Counting objects: 94% (133/141)[K remote: Counting objects: 95% (134/141)[K remote: Counting objects: 96% (136/141)[K remote: Counting objects: 97% (137/141)[K
remote: Counting objects: 98% (139/141)[K
remote: Counting objects: 99% (140/141)[K remote: Counting objects: 100% (141/141)[K remote: Counting objects: 100% (141/141), done.[K
remote: Compressing objects: 0% (1/115)[K
remote: Compressing objects: 1% (2/115)[K remote: Compressing objects: 2% (3/115)[K remote: Compressing objects: 3% (4/115)[K remote: Compressing objects: 4% (5/115)[K remote: Compressing objects: 5% (6/115)[K remote: Compressing objects: 6% (7/115)[K
remote: Compressing objects: 7% (9/115)[K
remote: Compressing objects: 8% (10/115)[K remote: Compressing objects: 9% (11/115)[K
remote: Compressing objects: 10% (12/115)[K
remote: Compressing objects: 11% (13/115)[K
remote: Compressing objects: 12% (14/115)[K
remote: Compressing objects: 13% (15/115)[K remote: Compressing objects: 14% (17/115)[K remote: Compressing objects: 15% (18/115)[K remote: Compressing objects: 16% (19/115)[K remote: Compressing objects: 17% (20/115)[K remote: Compressing objects: 18% (21/115)[K remote: Compressing objects: 19% (22/115)[K remote: Compressing objects: 20% (23/115)[K remote: Compressing objects: 21% (25/115)[K remote: Compressing objects: 22% (26/115)[K remote: Compressing objects: 23% (27/115)[K remote: Compressing objects: 24% (28/115)[K remote: Compressing objects: 25% (29/115)[K remote: Compressing objects: 26% (30/115)[K remote: Compressing objects: 27% (32/115)[K remote: Compressing objects: 28% (33/115)[K remote: Compressing objects: 29% (34/115)[K remote: Compressing objects: 30% (35/115)[K remote: Compressing objects: 31% (36/115)[K remote: Compressing objects: 32% (37/115)[K remote: Compressing objects: 33% (38/115)[K remote: Compressing objects: 34% (40/115)[K
remote: Compressing objects: 35% (41/115)[K
remote: Compressing objects: 36% (42/115)[K remote: Compressing objects: 37% (43/115)[K remote: Compressing objects: 38% (44/115)[K remote: Compressing objects: 39% (45/115)[K remote: Compressing objects: 40% (46/115)[K remote: Compressing objects: 41% (48/115)[K remote: Compressing objects: 42% (49/115)[K remote: Compressing objects: 43% (50/115)[K remote: Compressing objects: 44% (51/115)[K remote: Compressing objects: 45% (52/115)[K remote: Compressing objects: 46% (53/115)[K remote: Compressing objects: 47% (55/115)[K remote: Compressing objects: 48% (56/115)[K remote: Compressing objects: 49% (57/115)[K remote: Compressing objects: 50% (58/115)[K remote: Compressing objects: 51% (59/115)[K remote: Compressing objects: 52% (60/115)[K remote: Compressing objects: 53% (61/115)[K remote: Compressing objects: 54% (63/115)[K remote: Compressing objects: 55% (64/115)[K remote: Compressing objects: 56% (65/115)[K remote: Compressing objects: 57% (66/115)[K remote: Compressing objects: 58% (67/115)[K remote: Compressing objects: 59% (68/115)[K remote: Compressing objects: 60% (69/115)[K remote: Compressing objects: 61% (71/115)[K remote: Compressing objects: 62% (72/115)[K remote: Compressing objects: 63% (73/115)[K remote: Compressing objects: 64% (74/115)[K remote: Compressing objects: 65% (75/115)[K remote: Compressing objects: 66% (76/115)[K remote: Compressing objects: 67% (78/115)[K remote: Compressing objects: 68% (79/115)[K remote: Compressing objects: 69% (80/115)[K remote: Compressing objects: 70% (81/115)[K remote: Compressing objects: 71% (82/115)[K remote: Compressing objects: 72% (83/115)[K remote: Compressing objects: 73% (84/115)[K remote: Compressing objects: 74% (86/115)[K remote: Compressing objects: 75% (87/115)[K remote: Compressing objects: 76% (88/115)[K remote: Compressing objects: 77% (89/115)[K remote: Compressing objects: 78% (90/115)[K remote: Compressing objects: 79% (91/115)[K remote: Compressing objects: 80% (92/115)[K remote: Compressing objects: 81% (94/115)[K remote: Compressing objects: 82% (95/115)[K remote: Compressing objects: 83% (96/115)[K remote: Compressing objects: 84% (97/115)[K remote: Compressing objects: 85% (98/115)[K remote: Compressing objects: 86% (99/115)[K remote: Compressing objects: 87% (101/115)[K remote: Compressing objects: 88% (102/115)[K remote: Compressing objects: 89% (103/115)[K remote: Compressing objects: 90% (104/115)[K remote: Compressing objects: 91% (105/115)[K remote: Compressing objects: 92% (106/115)[K remote: Compressing objects: 93% (107/115)[K remote: Compressing objects: 94% (109/115)[K remote: Compressing objects: 95% (110/115)[K remote: Compressing objects: 96% (111/115)[K remote: Compressing objects: 97% (112/115)[K remote: Compressing objects: 98% (113/115)[K remote: Compressing objects: 99% (114/115)[K remote: Compressing objects: 100% (115/115)[K remote: Compressing objects: 100% (115/115), done.[K
Receiving objects: 0% (1/406)
Receiving objects: 0% (2/406), 3.58 MiB | 3.49 MiB/s
Receiving objects: 0% (3/406), 7.50 MiB | 3.64 MiB/s
Receiving objects: 0% (4/406), 10.91 MiB | 3.52 MiB/s
Receiving objects: 0% (4/406), 13.38 MiB | 3.25 MiB/s
Receiving objects: 0% (4/406), 16.37 MiB | 3.19 MiB/s
Receiving objects: 1% (5/406), 17.36 MiB | 2.98 MiB/s
Receiving objects: 2% (9/406), 17.36 MiB | 2.98 MiB/s
Receiving objects: 2% (11/406), 18.32 MiB | 2.78 MiB/s
Receiving objects: 2% (12/406), 19.92 MiB | 2.29 MiB/s
Receiving objects: 2% (12/406), 21.36 MiB | 2.07 MiB/s
Receiving objects: 2% (12/406), 22.94 MiB | 1.69 MiB/s
Receiving objects: 2% (12/406), 24.57 MiB | 1.59 MiB/s
Receiving objects: 3% (13/406), 24.57 MiB | 1.59 MiB/s
Receiving objects: 3% (14/406), 26.98 MiB | 1.78 MiB/s
Receiving objects: 3% (14/406), 29.75 MiB | 1.96 MiB/s
Receiving objects: 3% (14/406), 31.61 MiB | 2.12 MiB/s
Receiving objects: 3% (15/406), 33.66 MiB | 2.16 MiB/s
Receiving objects: 3% (15/406), 35.47 MiB | 2.21 MiB/s
Receiving objects: 3% (15/406), 38.89 MiB | 2.21 MiB/s
Receiving objects: 3% (16/406), 42.68 MiB | 2.66 MiB/s
Receiving objects: 4% (17/406), 44.61 MiB | 2.86 MiB/s
Receiving objects: 4% (17/406), 45.92 MiB | 2.91 MiB/s
Receiving objects: 4% (17/406), 49.71 MiB | 3.32 MiB/s
Receiving objects: 4% (18/406), 53.49 MiB | 3.63 MiB/s
Receiving objects: 4% (19/406), 56.21 MiB | 3.36 MiB/s
Receiving objects: 4% (19/406), 58.43 MiB | 3.01 MiB/s
Receiving objects: 5% (21/406), 58.43 MiB | 3.01 MiB/s
Receiving objects: 5% (21/406), 61.68 MiB | 3.02 MiB/s
Receiving objects: 5% (21/406), 64.04 MiB | 2.71 MiB/s
Receiving objects: 5% (22/406), 66.81 MiB | 2.63 MiB/s
Receiving objects: 5% (22/406), 69.60 MiB | 2.80 MiB/s
Receiving objects: 6% (25/406), 69.60 MiB | 2.80 MiB/s
Receiving objects: 7% (29/406), 69.60 MiB | 2.80 MiB/s
Receiving objects: 7% (29/406), 73.45 MiB | 2.89 MiB/s
Receiving objects: 7% (32/406), 77.25 MiB | 3.26 MiB/s
Receiving objects: 8% (33/406), 77.25 MiB | 3.26 MiB/s
Receiving objects: 8% (33/406), 81.05 MiB | 3.33 MiB/s
Receiving objects: 8% (34/406), 84.83 MiB | 3.75 MiB/s
Receiving objects: 8% (35/406), 86.69 MiB | 3.77 MiB/s
Receiving objects: 9% (37/406), 86.69 MiB | 3.77 MiB/s Receiving objects: 10% (41/406), 86.69 MiB | 3.77 MiB/s
Receiving objects: 10% (44/406), 92.43 MiB | 3.78 MiB/s
Receiving objects: 11% (45/406), 94.27 MiB | 3.76 MiB/s
Receiving objects: 12% (49/406), 96.22 MiB | 3.77 MiB/s
Receiving objects: 13% (53/406), 96.22 MiB | 3.77 MiB/s Receiving objects: 14% (57/406), 96.22 MiB | 3.77 MiB/s Receiving objects: 15% (61/406), 96.22 MiB | 3.77 MiB/s Receiving objects: 16% (65/406), 96.22 MiB | 3.77 MiB/s
Receiving objects: 17% (70/406), 96.22 MiB | 3.77 MiB/s
Receiving objects: 17% (73/406), 98.10 MiB | 3.74 MiB/s
Receiving objects: 18% (74/406), 98.10 MiB | 3.74 MiB/s
Receiving objects: 18% (74/406), 101.26 MiB | 3.58 MiB/s
Receiving objects: 19% (78/406), 103.22 MiB | 3.59 MiB/s
Receiving objects: 20% (82/406), 103.22 MiB | 3.59 MiB/s Receiving objects: 21% (86/406), 103.22 MiB | 3.59 MiB/s Receiving objects: 22% (90/406), 103.22 MiB | 3.59 MiB/s
Receiving objects: 22% (93/406), 105.18 MiB | 3.59 MiB/s
Receiving objects: 23% (94/406), 107.12 MiB | 3.59 MiB/s
Receiving objects: 24% (98/406), 107.12 MiB | 3.59 MiB/s
Receiving objects: 24% (100/406), 108.48 MiB | 3.44 MiB/s
Receiving objects: 24% (100/406), 111.05 MiB | 3.18 MiB/s
Receiving objects: 25% (102/406), 111.05 MiB | 3.18 MiB/s
Receiving objects: 26% (106/406), 111.05 MiB | 3.18 MiB/s
Receiving objects: 27% (110/406), 111.05 MiB | 3.18 MiB/s
Receiving objects: 28% (114/406), 111.05 MiB | 3.18 MiB/s
Receiving objects: 29% (118/406), 111.05 MiB | 3.18 MiB/s Receiving objects: 30% (122/406), 112.33 MiB | 3.06 MiB/s Receiving objects: 31% (126/406), 112.33 MiB | 3.06 MiB/s
Receiving objects: 31% (128/406), 113.19 MiB | 2.99 MiB/s
Receiving objects: 31% (129/406), 114.94 MiB | 2.96 MiB/s
Receiving objects: 32% (130/406), 114.94 MiB | 2.96 MiB/s
Receiving objects: 33% (134/406), 116.81 MiB | 2.94 MiB/s
Receiving objects: 34% (139/406), 116.81 MiB | 2.94 MiB/s
Receiving objects: 35% (143/406), 116.81 MiB | 2.94 MiB/s
Receiving objects: 36% (147/406), 116.81 MiB | 2.94 MiB/s Receiving objects: 37% (151/406), 116.81 MiB | 2.94 MiB/s Receiving objects: 38% (155/406), 116.81 MiB | 2.94 MiB/s
Receiving objects: 39% (159/406), 116.81 MiB | 2.94 MiB/s
Receiving objects: 40% (163/406), 116.81 MiB | 2.94 MiB/s Receiving objects: 41% (167/406), 116.81 MiB | 2.94 MiB/s Receiving objects: 42% (171/406), 116.81 MiB | 2.94 MiB/s Receiving objects: 43% (175/406), 116.81 MiB | 2.94 MiB/s Receiving objects: 44% (179/406), 116.81 MiB | 2.94 MiB/s Receiving objects: 45% (183/406), 116.81 MiB | 2.94 MiB/s Receiving objects: 46% (187/406), 116.81 MiB | 2.94 MiB/s Receiving objects: 47% (191/406), 116.81 MiB | 2.94 MiB/s Receiving objects: 48% (195/406), 116.81 MiB | 2.94 MiB/s Receiving objects: 49% (199/406), 116.81 MiB | 2.94 MiB/s Receiving objects: 50% (203/406), 116.81 MiB | 2.94 MiB/s Receiving objects: 51% (208/406), 116.81 MiB | 2.94 MiB/s Receiving objects: 52% (212/406), 116.81 MiB | 2.94 MiB/s Receiving objects: 53% (216/406), 116.81 MiB | 2.94 MiB/s Receiving objects: 54% (220/406), 116.81 MiB | 2.94 MiB/s Receiving objects: 55% (224/406), 116.81 MiB | 2.94 MiB/s Receiving objects: 56% (228/406), 116.81 MiB | 2.94 MiB/s Receiving objects: 57% (232/406), 116.81 MiB | 2.94 MiB/s Receiving objects: 58% (236/406), 116.81 MiB | 2.94 MiB/s Receiving objects: 59% (240/406), 116.81 MiB | 2.94 MiB/s Receiving objects: 60% (244/406), 116.81 MiB | 2.94 MiB/s Receiving objects: 61% (248/406), 116.81 MiB | 2.94 MiB/s Receiving objects: 62% (252/406), 116.81 MiB | 2.94 MiB/s Receiving objects: 63% (256/406), 116.81 MiB | 2.94 MiB/s Receiving objects: 64% (260/406), 116.81 MiB | 2.94 MiB/s Receiving objects: 65% (264/406), 116.81 MiB | 2.94 MiB/s Receiving objects: 66% (268/406), 116.81 MiB | 2.94 MiB/s Receiving objects: 67% (273/406), 116.81 MiB | 2.94 MiB/s Receiving objects: 68% (277/406), 116.81 MiB | 2.94 MiB/s Receiving objects: 69% (281/406), 116.81 MiB | 2.94 MiB/s Receiving objects: 70% (285/406), 116.81 MiB | 2.94 MiB/s Receiving objects: 71% (289/406), 116.81 MiB | 2.94 MiB/s Receiving objects: 72% (293/406), 116.81 MiB | 2.94 MiB/s Receiving objects: 73% (297/406), 116.81 MiB | 2.94 MiB/s Receiving objects: 74% (301/406), 116.81 MiB | 2.94 MiB/s Receiving objects: 75% (305/406), 116.81 MiB | 2.94 MiB/s Receiving objects: 76% (309/406), 116.81 MiB | 2.94 MiB/s Receiving objects: 77% (313/406), 116.81 MiB | 2.94 MiB/s Receiving objects: 78% (317/406), 116.81 MiB | 2.94 MiB/s Receiving objects: 79% (321/406), 116.81 MiB | 2.94 MiB/s Receiving objects: 80% (325/406), 116.81 MiB | 2.94 MiB/s Receiving objects: 81% (329/406), 116.81 MiB | 2.94 MiB/s Receiving objects: 82% (333/406), 116.81 MiB | 2.94 MiB/s Receiving objects: 83% (337/406), 116.81 MiB | 2.94 MiB/s Receiving objects: 84% (342/406), 116.81 MiB | 2.94 MiB/s Receiving objects: 85% (346/406), 116.81 MiB | 2.94 MiB/s Receiving objects: 86% (350/406), 116.81 MiB | 2.94 MiB/s Receiving objects: 87% (354/406), 116.81 MiB | 2.94 MiB/s Receiving objects: 88% (358/406), 116.81 MiB | 2.94 MiB/s
Receiving objects: 88% (360/406), 119.14 MiB | 2.62 MiB/s
Receiving objects: 88% (360/406), 122.64 MiB | 2.93 MiB/s
Receiving objects: 88% (360/406), 126.48 MiB | 3.11 MiB/s
Receiving objects: 88% (360/406), 129.00 MiB | 3.09 MiB/s
Receiving objects: 89% (362/406), 129.00 MiB | 3.09 MiB/s
Receiving objects: 89% (363/406), 132.79 MiB | 3.17 MiB/s
Receiving objects: 89% (363/406), 136.70 MiB | 3.48 MiB/s
Receiving objects: 89% (363/406), 139.84 MiB | 3.31 MiB/s
Receiving objects: 89% (363/406), 143.14 MiB | 3.39 MiB/s
Receiving objects: 89% (363/406), 146.93 MiB | 3.49 MiB/s
Receiving objects: 90% (366/406), 146.93 MiB | 3.49 MiB/s
Receiving objects: 90% (368/406), 150.72 MiB | 3.50 MiB/s
Receiving objects: 90% (368/406), 154.51 MiB | 3.50 MiB/s
Receiving objects: 91% (370/406), 154.51 MiB | 3.50 MiB/s
Receiving objects: 91% (373/406), 158.29 MiB | 3.77 MiB/s
Receiving objects: 91% (373/406), 162.08 MiB | 3.77 MiB/s
Receiving objects: 91% (373/406), 165.89 MiB | 3.77 MiB/s
Receiving objects: 91% (373/406), 169.69 MiB | 3.78 MiB/s
Receiving objects: 92% (374/406), 171.59 MiB | 3.78 MiB/s
Receiving objects: 92% (376/406), 177.27 MiB | 3.77 MiB/s
Receiving objects: 92% (376/406), 181.00 MiB | 3.76 MiB/s
Receiving objects: 92% (376/406), 184.79 MiB | 3.76 MiB/s
Receiving objects: 93% (378/406), 184.79 MiB | 3.76 MiB/s
Receiving objects: 94% (382/406), 186.74 MiB | 3.77 MiB/s
Receiving objects: 95% (386/406), 188.58 MiB | 3.76 MiB/s
Receiving objects: 95% (387/406), 192.37 MiB | 3.76 MiB/s
Receiving objects: 95% (388/406), 194.32 MiB | 3.76 MiB/s
Receiving objects: 95% (388/406), 200.04 MiB | 3.77 MiB/s
Receiving objects: 95% (388/406), 203.84 MiB | 3.77 MiB/s
Receiving objects: 95% (388/406), 207.62 MiB | 3.77 MiB/s
Receiving objects: 95% (388/406), 210.38 MiB | 3.54 MiB/s
Receiving objects: 96% (390/406), 210.38 MiB | 3.54 MiB/s
Receiving objects: 96% (393/406), 212.25 MiB | 3.51 MiB/s
Receiving objects: 97% (394/406), 212.25 MiB | 3.51 MiB/s
Receiving objects: 97% (394/406), 218.10 MiB | 3.54 MiB/s
Receiving objects: 97% (394/406), 221.87 MiB | 3.52 MiB/s
Receiving objects: 97% (394/406), 225.63 MiB | 3.52 MiB/s
Receiving objects: 97% (394/406), 229.47 MiB | 3.77 MiB/s
Receiving objects: 97% (394/406), 233.26 MiB | 3.76 MiB/s
Receiving objects: 97% (394/406), 235.18 MiB | 3.75 MiB/s
Receiving objects: 98% (398/406), 237.16 MiB | 3.78 MiB/s
remote: Total 406 (delta 72), reused 69 (delta 26), pack-reused 265[K
Receiving objects: 99% (402/406), 237.16 MiB | 3.78 MiB/s
Receiving objects: 100% (406/406), 237.16 MiB | 3.78 MiB/s Receiving objects: 100% (406/406), 237.89 MiB | 3.23 MiB/s, done.
Resolving deltas: 0% (0/124)
Resolving deltas: 1% (2/124) Resolving deltas: 2% (3/124) Resolving deltas: 3% (4/124) Resolving deltas: 4% (6/124) Resolving deltas: 5% (7/124) Resolving deltas: 7% (9/124) Resolving deltas: 9% (12/124) Resolving deltas: 13% (17/124) Resolving deltas: 16% (20/124) Resolving deltas: 19% (24/124) Resolving deltas: 22% (28/124) Resolving deltas: 25% (31/124) Resolving deltas: 28% (35/124) Resolving deltas: 31% (39/124) Resolving deltas: 32% (40/124) Resolving deltas: 36% (45/124) Resolving deltas: 37% (46/124) Resolving deltas: 39% (49/124) Resolving deltas: 40% (50/124) Resolving deltas: 41% (51/124) Resolving deltas: 43% (54/124) Resolving deltas: 58% (72/124) Resolving deltas: 64% (80/124) Resolving deltas: 68% (85/124) Resolving deltas: 72% (90/124) Resolving deltas: 75% (94/124) Resolving deltas: 80% (100/124) Resolving deltas: 81% (101/124) Resolving deltas: 83% (103/124) Resolving deltas: 84% (105/124) Resolving deltas: 85% (106/124) Resolving deltas: 86% (107/124) Resolving deltas: 100% (124/124) Resolving deltas: 100% (124/124), done.
/opt/home/k8sworker/ci-ai/cibuilds/ov-notebook/OVNotebookOps-609/.workspace/scm/ov-notebook/notebooks/280-depth-anything/Depth-Anything
DEPRECATION: pytorch-lightning 1.6.5 has a non-standard dependency specifier torch>=1.8.*. pip 24.1 will enforce this behaviour change. A possible replacement is to upgrade to a newer version of pytorch-lightning or contact the author to suggest that they release a version with a conforming dependency specifiers. Discussion can be found at https://github.com/pypa/pip/issues/12063
Note: you may need to restart the kernel to use updated packages.
DEPRECATION: pytorch-lightning 1.6.5 has a non-standard dependency specifier torch>=1.8.*. pip 24.1 will enforce this behaviour change. A possible replacement is to upgrade to a newer version of pytorch-lightning or contact the author to suggest that they release a version with a conforming dependency specifiers. Discussion can be found at https://github.com/pypa/pip/issues/12063
Note: you may need to restart the kernel to use updated packages.
DEPRECATION: pytorch-lightning 1.6.5 has a non-standard dependency specifier torch>=1.8.*. pip 24.1 will enforce this behaviour change. A possible replacement is to upgrade to a newer version of pytorch-lightning or contact the author to suggest that they release a version with a conforming dependency specifiers. Discussion can be found at https://github.com/pypa/pip/issues/12063
/bin/bash: =: No such file or directory
Note: you may need to restart the kernel to use updated packages.
DEPRECATION: pytorch-lightning 1.6.5 has a non-standard dependency specifier torch>=1.8.*. pip 24.1 will enforce this behaviour change. A possible replacement is to upgrade to a newer version of pytorch-lightning or contact the author to suggest that they release a version with a conforming dependency specifiers. Discussion can be found at https://github.com/pypa/pip/issues/12063
ERROR: pip's dependency resolver does not currently take into account all the packages that are installed. This behaviour is the source of the following dependency conflicts.
pyannote-audio 2.0.1 requires torchaudio<1.0,>=0.10, but you have torchaudio 2.2.0+cpu which is incompatible.
tokenizers 0.14.1 requires huggingface_hub<0.18,>=0.16.4, but you have huggingface-hub 0.20.3 which is incompatible.
Note: you may need to restart the kernel to use updated packages.
Load and run PyTorch model¶
To be able run PyTorch model on CPU, we should disable xformers attention optimizations first.
attention_file_path = Path("./torchhub/facebookresearch_dinov2_main/dinov2/layers/attention.py")
orig_attention_path = attention_file_path.parent / ("orig_" + attention_file_path.name)
if not orig_attention_path.exists():
attention_file_path.rename(orig_attention_path)
with orig_attention_path.open("r") as f:
data = f.read()
data = data.replace("XFORMERS_AVAILABLE = True", "XFORMERS_AVAILABLE = False")
with attention_file_path.open("w") as out_f:
out_f.write(data)
DepthAnything.from_pretrained
method creates PyTorch model class
instance and load model weights. There are 3 available models in
repository depends on VIT encoder size: * Depth-Anything-ViT-Small
(24.8M) * Depth-Anything-ViT-Base (97.5M) * Depth-Anything-ViT-Large
(335.3M)
We will use Depth-Anything-ViT-Small
, but the same steps for running
model and converting to OpenVINO are applicable for other models from
DepthAnything family.
from depth_anything.dpt import DepthAnything
encoder = 'vits' # can also be 'vitb' or 'vitl'
model_id = 'depth_anything_{:}14'.format(encoder)
depth_anything = DepthAnything.from_pretrained(f'LiheYoung/{model_id}')
xFormers not available
xFormers not available
Prepare input data¶
import urllib.request
from PIL import Image
urllib.request.urlretrieve(
url='https://raw.githubusercontent.com/openvinotoolkit/openvino_notebooks/main/notebooks/utils/notebook_utils.py',
filename='notebook_utils.py'
)
from notebook_utils import download_file
download_file("https://github.com/openvinotoolkit/openvino_notebooks/assets/29454499/3f779fc1-c1b2-4dec-915a-64dae510a2bb", "furseal.png")
Image.open("furseal.png").resize((600, 400))
furseal.png: 0%| | 0.00/2.55M [00:00<?, ?B/s]
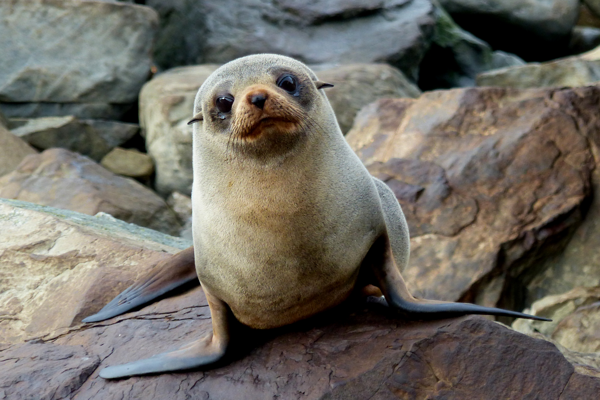
for simplicity of usage, model authors provide helper functions for preprocessing input image. The main conditions are that image size should be divisible on 14 (size of vit patch) and normalized in [0, 1] range.
from depth_anything.util.transform import Resize, NormalizeImage, PrepareForNet
from torchvision.transforms import Compose
import cv2
import torch
transform = Compose([
Resize(
width=518,
height=518,
resize_target=False,
ensure_multiple_of=14,
resize_method='lower_bound',
image_interpolation_method=cv2.INTER_CUBIC,
),
NormalizeImage(mean=[0.485, 0.456, 0.406], std=[0.229, 0.224, 0.225]),
PrepareForNet(),
])
image = cv2.cvtColor(cv2.imread('furseal.png'), cv2.COLOR_BGR2RGB) / 255.0
h, w = image.shape[:-1]
image = transform({'image': image})['image']
image = torch.from_numpy(image).unsqueeze(0)
Run model inference¶
Preprocessed image passed to model forward and model returns depth map
in format B
x H
x W
, where B
is input batch size, H
is preprocessed image height, W
is preprocessed image width.
# depth shape: 1xHxW
depth = depth_anything(image)
After image processing finished, we can resize depth map into original image size and prepare it for visualization.
import torch.nn.functional as F
import numpy as np
depth = F.interpolate(depth[None], (h, w), mode='bilinear', align_corners=False)[0, 0]
depth = (depth - depth.min()) / (depth.max() - depth.min()) * 255.0
depth = depth.cpu().detach().numpy().astype(np.uint8)
depth_color = cv2.applyColorMap(depth, cv2.COLORMAP_INFERNO)
from matplotlib import pyplot as plt
plt.imshow(depth_color[:, :, ::-1]);
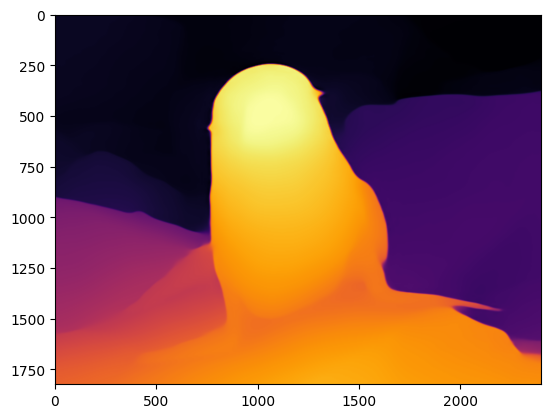
Convert Model to OpenVINO IR format¶
OpenVINO supports PyTorch models via conversion to OpenVINO Intermediate
Representation (IR). OpenVINO model conversion API should be used for
these purposes. ov.convert_model
function accepts original PyTorch
model instance and example input for tracing and returns ov.Model
representing this model in OpenVINO framework. Converted model can be
used for saving on disk using ov.save_model
function or directly
loading on device using core.complie_model
.
import openvino as ov
OV_DEPTH_ANYTHING_PATH = Path(f'{model_id}.xml')
if not OV_DEPTH_ANYTHING_PATH.exists():
ov_model = ov.convert_model(depth_anything, example_input=image, input=[1, 3, 518, 518])
ov.save_model(ov_model, OV_DEPTH_ANYTHING_PATH)
/opt/home/k8sworker/ci-ai/cibuilds/ov-notebook/OVNotebookOps-609/.workspace/scm/ov-notebook/notebooks/280-depth-anything/Depth-Anything/torchhub/facebookresearch_dinov2_main/dinov2/layers/patch_embed.py:73: TracerWarning: Converting a tensor to a Python boolean might cause the trace to be incorrect. We can't record the data flow of Python values, so this value will be treated as a constant in the future. This means that the trace might not generalize to other inputs!
assert H % patch_H == 0, f"Input image height {H} is not a multiple of patch height {patch_H}"
/opt/home/k8sworker/ci-ai/cibuilds/ov-notebook/OVNotebookOps-609/.workspace/scm/ov-notebook/notebooks/280-depth-anything/Depth-Anything/torchhub/facebookresearch_dinov2_main/dinov2/layers/patch_embed.py:74: TracerWarning: Converting a tensor to a Python boolean might cause the trace to be incorrect. We can't record the data flow of Python values, so this value will be treated as a constant in the future. This means that the trace might not generalize to other inputs!
assert W % patch_W == 0, f"Input image width {W} is not a multiple of patch width: {patch_W}"
/opt/home/k8sworker/ci-ai/cibuilds/ov-notebook/OVNotebookOps-609/.workspace/scm/ov-notebook/notebooks/280-depth-anything/Depth-Anything/torchhub/facebookresearch_dinov2_main/vision_transformer.py:183: TracerWarning: Converting a tensor to a Python boolean might cause the trace to be incorrect. We can't record the data flow of Python values, so this value will be treated as a constant in the future. This means that the trace might not generalize to other inputs!
if npatch == N and w == h:
/opt/home/k8sworker/ci-ai/cibuilds/ov-notebook/OVNotebookOps-609/.workspace/scm/ov-notebook/notebooks/280-depth-anything/Depth-Anything/depth_anything/dpt.py:133: TracerWarning: Converting a tensor to a Python integer might cause the trace to be incorrect. We can't record the data flow of Python values, so this value will be treated as a constant in the future. This means that the trace might not generalize to other inputs!
out = F.interpolate(out, (int(patch_h * 14), int(patch_w * 14)), mode="bilinear", align_corners=True)
Run OpenVINO model inference¶
Now, we are ready to run OpenVINO model
Select inference device¶
For starting work, please select inference device from dropdown list.
import ipywidgets as widgets
core = ov.Core()
device = widgets.Dropdown(
options=core.available_devices + ["AUTO"],
value="AUTO",
description="Device:",
disabled=False,
)
device
Dropdown(description='Device:', index=1, options=('CPU', 'AUTO'), value='AUTO')
compiled_model = core.compile_model(OV_DEPTH_ANYTHING_PATH, device.value)
Run inference on image¶
res = compiled_model(image)[0]
depth = res[0]
depth = cv2.resize(depth, (w, h))
depth = (depth - depth.min()) / (depth.max() - depth.min()) * 255.0
depth = depth.astype(np.uint8)
depth_color = cv2.applyColorMap(depth, cv2.COLORMAP_INFERNO)
plt.imshow(depth_color[:, :, ::-1]);
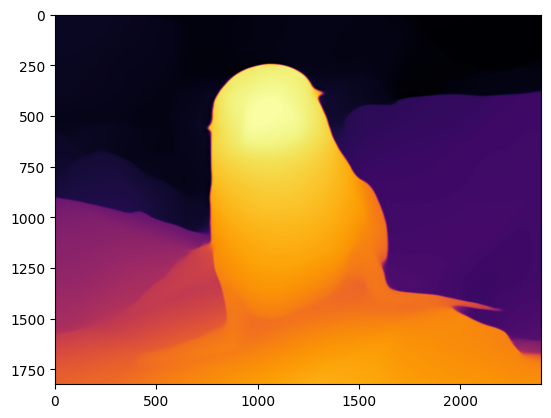
Run inference on video¶
download_file("https://storage.openvinotoolkit.org/repositories/openvino_notebooks/data/data/video/Coco%20Walking%20in%20Berkeley.mp4", "./Coco Walking in Berkeley.mp4")
VIDEO_FILE = "./Coco Walking in Berkeley.mp4"
# Number of seconds of input video to process. Set `NUM_SECONDS` to 0 to process
# the full video.
NUM_SECONDS = 4
# Set `ADVANCE_FRAMES` to 1 to process every frame from the input video
# Set `ADVANCE_FRAMES` to 2 to process every second frame. This reduces
# the time it takes to process the video.
ADVANCE_FRAMES = 2
# Set `SCALE_OUTPUT` to reduce the size of the result video
# If `SCALE_OUTPUT` is 0.5, the width and height of the result video
# will be half the width and height of the input video.
SCALE_OUTPUT = 0.5
# The format to use for video encoding. The 'vp09` is slow,
# but it works on most systems.
# Try the `THEO` encoding if you have FFMPEG installed.
# FOURCC = cv2.VideoWriter_fourcc(*"THEO")
FOURCC = cv2.VideoWriter_fourcc(*"vp09")
# Create Path objects for the input video and the result video.
output_directory = Path("output")
output_directory.mkdir(exist_ok=True)
result_video_path = output_directory / f"{Path(VIDEO_FILE).stem}_depth_anything.mp4"
Coco Walking in Berkeley.mp4: 0%| | 0.00/877k [00:00<?, ?B/s]
cap = cv2.VideoCapture(str(VIDEO_FILE))
ret, image = cap.read()
if not ret:
raise ValueError(f"The video at {VIDEO_FILE} cannot be read.")
input_fps = cap.get(cv2.CAP_PROP_FPS)
input_video_frame_height, input_video_frame_width = image.shape[:2]
target_fps = input_fps / ADVANCE_FRAMES
target_frame_height = int(input_video_frame_height * SCALE_OUTPUT)
target_frame_width = int(input_video_frame_width * SCALE_OUTPUT)
cap.release()
print(
f"The input video has a frame width of {input_video_frame_width}, "
f"frame height of {input_video_frame_height} and runs at {input_fps:.2f} fps"
)
print(
"The output video will be scaled with a factor "
f"{SCALE_OUTPUT}, have width {target_frame_width}, "
f" height {target_frame_height}, and run at {target_fps:.2f} fps"
)
The input video has a frame width of 640, frame height of 360 and runs at 30.00 fps
The output video will be scaled with a factor 0.5, have width 320, height 180, and run at 15.00 fps
def normalize_minmax(data):
"""Normalizes the values in `data` between 0 and 1"""
return (data - data.min()) / (data.max() - data.min())
def convert_result_to_image(result, colormap="viridis"):
"""
Convert network result of floating point numbers to an RGB image with
integer values from 0-255 by applying a colormap.
`result` is expected to be a single network result in 1,H,W shape
`colormap` is a matplotlib colormap.
See https://matplotlib.org/stable/tutorials/colors/colormaps.html
"""
result = result.squeeze(0)
result = normalize_minmax(result)
result = result * 255
result = result.astype(np.uint8)
result = cv2.applyColorMap(result, cv2.COLORMAP_INFERNO)[:, :, ::-1]
return result
def to_rgb(image_data) -> np.ndarray:
"""
Convert image_data from BGR to RGB
"""
return cv2.cvtColor(image_data, cv2.COLOR_BGR2RGB)
import time
from IPython.display import (
HTML,
FileLink,
Pretty,
ProgressBar,
Video,
clear_output,
display,
)
# Initialize variables.
input_video_frame_nr = 0
start_time = time.perf_counter()
total_inference_duration = 0
# Open the input video
cap = cv2.VideoCapture(str(VIDEO_FILE))
# Create a result video.
out_video = cv2.VideoWriter(
str(result_video_path),
FOURCC,
target_fps,
(target_frame_width * 2, target_frame_height),
)
num_frames = int(NUM_SECONDS * input_fps)
total_frames = cap.get(cv2.CAP_PROP_FRAME_COUNT) if num_frames == 0 else num_frames
progress_bar = ProgressBar(total=total_frames)
progress_bar.display()
try:
while cap.isOpened():
ret, image = cap.read()
if not ret:
cap.release()
break
if input_video_frame_nr >= total_frames:
break
h, w = image.shape[:-1]
input_image = cv2.cvtColor(image, cv2.COLOR_BGR2RGB) / 255.0
input_image = transform({'image': input_image})['image']
# Reshape the image to network input shape NCHW.
input_image = np.expand_dims(input_image, 0)
# Do inference.
inference_start_time = time.perf_counter()
result = compiled_model(input_image)[0]
inference_stop_time = time.perf_counter()
inference_duration = inference_stop_time - inference_start_time
total_inference_duration += inference_duration
if input_video_frame_nr % (10 * ADVANCE_FRAMES) == 0:
clear_output(wait=True)
progress_bar.display()
# input_video_frame_nr // ADVANCE_FRAMES gives the number of
# Frames that have been processed by the network.
display(
Pretty(
f"Processed frame {input_video_frame_nr // ADVANCE_FRAMES}"
f"/{total_frames // ADVANCE_FRAMES}. "
f"Inference time per frame: {inference_duration:.2f} seconds "
f"({1/inference_duration:.2f} FPS)"
)
)
# Transform the network result to a RGB image.
result_frame = to_rgb(convert_result_to_image(result))
# Resize the image and the result to a target frame shape.
result_frame = cv2.resize(result_frame, (target_frame_width, target_frame_height))
image = cv2.resize(image, (target_frame_width, target_frame_height))
# Put the image and the result side by side.
stacked_frame = np.hstack((image, result_frame))
# Save a frame to the video.
out_video.write(stacked_frame)
input_video_frame_nr = input_video_frame_nr + ADVANCE_FRAMES
cap.set(1, input_video_frame_nr)
progress_bar.progress = input_video_frame_nr
progress_bar.update()
except KeyboardInterrupt:
print("Processing interrupted.")
finally:
clear_output()
processed_frames = num_frames // ADVANCE_FRAMES
out_video.release()
cap.release()
end_time = time.perf_counter()
duration = end_time - start_time
print(
f"Processed {processed_frames} frames in {duration:.2f} seconds. "
f"Total FPS (including video processing): {processed_frames/duration:.2f}."
f"Inference FPS: {processed_frames/total_inference_duration:.2f} "
)
print(f"Video saved to '{str(result_video_path)}'.")
Processed 60 frames in 13.14 seconds. Total FPS (including video processing): 4.57.Inference FPS: 10.78
Video saved to 'output/Coco Walking in Berkeley_depth_anything.mp4'.
video = Video(result_video_path, width=800, embed=True)
if not result_video_path.exists():
plt.imshow(stacked_frame)
raise ValueError("OpenCV was unable to write the video file. Showing one video frame.")
else:
print(f"Showing video saved at\n{result_video_path.resolve()}")
print(
"If you cannot see the video in your browser, please click on the "
"following link to download the video "
)
video_link = FileLink(result_video_path)
video_link.html_link_str = "<a href='%s' download>%s</a>"
display(HTML(video_link._repr_html_()))
display(video)
Showing video saved at
/opt/home/k8sworker/ci-ai/cibuilds/ov-notebook/OVNotebookOps-609/.workspace/scm/ov-notebook/notebooks/280-depth-anything/Depth-Anything/output/Coco Walking in Berkeley_depth_anything.mp4
If you cannot see the video in your browser, please click on the following link to download the video
Interactive demo¶
You can apply model on own images. You can move the slider on the resulting image to switch between the original image and the depth map view.
import gradio as gr
import cv2
import numpy as np
import os
import tempfile
from gradio_imageslider import ImageSlider
css = """
#img-display-container {
max-height: 100vh;
}
#img-display-input {
max-height: 80vh;
}
#img-display-output {
max-height: 80vh;
}
"""
title = "# Depth Anything with OpenVINO"
def predict_depth(model, image):
return model(image)[0]
with gr.Blocks(css=css) as demo:
gr.Markdown(title)
gr.Markdown("### Depth Prediction demo")
gr.Markdown("You can slide the output to compare the depth prediction with input image")
with gr.Row():
input_image = gr.Image(label="Input Image", type='numpy', elem_id='img-display-input')
depth_image_slider = ImageSlider(label="Depth Map with Slider View", elem_id='img-display-output', position=0)
raw_file = gr.File(label="16-bit raw depth (can be considered as disparity)")
submit = gr.Button("Submit")
def on_submit(image):
original_image = image.copy()
h, w = image.shape[:2]
image = cv2.cvtColor(image, cv2.COLOR_BGR2RGB) / 255.0
image = transform({'image': image})['image']
image = np.expand_dims(image, 0)
depth = predict_depth(compiled_model, image)
depth = cv2.resize(depth[0], (w, h), interpolation=cv2.INTER_LINEAR)
raw_depth = Image.fromarray(depth.astype('uint16'))
tmp = tempfile.NamedTemporaryFile(suffix='.png', delete=False)
raw_depth.save(tmp.name)
depth = (depth - depth.min()) / (depth.max() - depth.min()) * 255.0
depth = depth.astype(np.uint8)
colored_depth = cv2.applyColorMap(depth, cv2.COLORMAP_INFERNO)[:, :, ::-1]
return [(original_image, colored_depth), tmp.name]
submit.click(on_submit, inputs=[input_image], outputs=[depth_image_slider, raw_file])
example_files = os.listdir('assets/examples')
example_files.sort()
example_files = [os.path.join('assets/examples', filename) for filename in example_files]
examples = gr.Examples(examples=example_files, inputs=[input_image], outputs=[depth_image_slider, raw_file], fn=on_submit, cache_examples=False)
if __name__ == '__main__':
try:
demo.queue().launch(debug=False)
except Exception:
demo.queue().launch(share=True, debug=False)
# if you are launching remotely, specify server_name and server_port
# demo.launch(server_name='your server name', server_port='server port in int')
# Read more in the docs: https://gradio.app/docs/
Running on local URL: http://127.0.0.1:7860 To create a public link, set share=True in launch().