Stable Diffusion v2.1 using Optimum-Intel OpenVINO and multiple Intel Hardware#
This Jupyter notebook can be launched after a local installation only.
This notebook will provide you a way to see different precision models performing in different hardware. This notebook was done for showing case the use of Optimum-Intel-OpenVINO and it is not optimized for running multiple times.
Table of contents:
Installation Instructions#
This is a self-contained example that relies solely on its own code.
We recommend running the notebook in a virtual environment. You only need a Jupyter server to start. For details, please refer to Installation Guide.
Optimum Intel is the interface between the Transformers and Diffusers libraries and the different tools and libraries provided by Intel to accelerate end-to-end pipelines on Intel architectures. More details in this repository.
Note: We suggest you to create a different environment and run the following installation command there.
%pip install -q "git+https://github.com/huggingface/optimum-intel.git" "diffusers>=0.25.0" "openvino>=2024.4.0" "ipywidgets" "transformers>=4.33.0" "torch>=2.1" --extra-index-url https://download.pytorch.org/whl/cpu
Stable Diffusion pipeline should brings 6 elements together, a text encoder model with a tokenizer, a UNet model with and scheduler, and an Autoencoder with Decoder and Encoder models.
image#
The base model used for this example is the stabilityai/stable-diffusion-2-1-base. This model was converted to OpenVINO format, for accelerated inference on CPU or Intel GPU with OpenVINO’s integration into Optimum.
import warnings
warnings.filterwarnings("ignore")
Showing Info Available Devices#
The available_devices
property shows the available devices in your
system. The “FULL_DEVICE_NAME” option to ie.get_property()
shows the
name of the device. Check what is the ID name for the discrete GPU, if
you have integrated GPU (iGPU) and discrete GPU (dGPU), it will show
device_name="GPU.0"
for iGPU and device_name="GPU.1"
for dGPU.
If you just have either an iGPU or dGPU that will be assigned to
"GPU"
import openvino as ov
import openvino.properties as props
core = ov.Core()
devices = core.available_devices
for device in devices:
device_name = core.get_property(device, props.device.full_name)
print(f"{device}: {device_name}")
CPU: Intel(R) Core(TM) Ultra 7 155H
GPU: Intel(R) Arc(TM) Graphics (iGPU)
NPU: Intel(R) AI Boost
Configure Inference Pipeline#
Select device from dropdown list for running inference using OpenVINO.
import requests
r = requests.get(
url="https://raw.githubusercontent.com/openvinotoolkit/openvino_notebooks/latest/utils/notebook_utils.py",
)
open("notebook_utils.py", "w").write(r.text)
from notebook_utils import device_widget
device = device_widget("CPU")
device
Dropdown(description='Device:', index=1, options=('CPU', 'GPU', 'NPU', 'AUTO'), value='GPU')
Convert model using Optimum#
from pathlib import Path
name = "stabilityai/stable-diffusion-2-1-base"
model_dir = Path(name.split("/")[-1])
if not model_dir.exists():
!optimum-cli export openvino -m {name} {model_dir}
Run model using OVStableDiffusionPipeline
#
from optimum.intel.openvino import OVStableDiffusionPipeline
# download and converted SD v2.1 model from Hugging Face Hub
ov_pipe = OVStableDiffusionPipeline.from_pretrained(model_dir, compile=False, device=device.value)
ov_pipe.reshape(batch_size=1, height=512, width=512, num_images_per_prompt=1)
ov_pipe.compile()
import gc
# Generate an image.
prompt = "red car in snowy forest, epic vista, beautiful landscape, 4k, 8k"
output_ov = ov_pipe(prompt, num_inference_steps=17, output_type="pil").images[0]
output_ov.save("image.png")
output_ov
0%| | 0/18 [00:00<?, ?it/s]
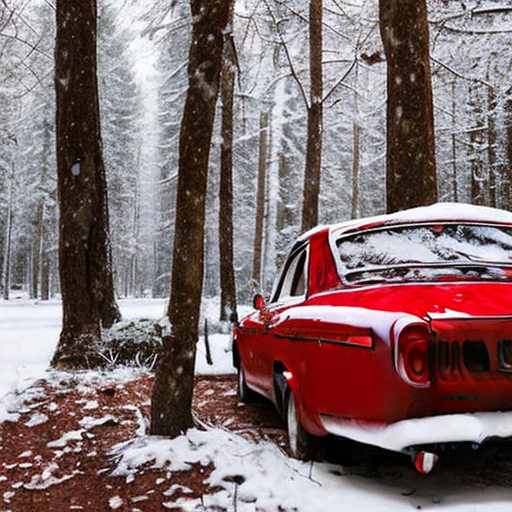
del ov_pipe
gc.collect();