person-vehicle-bike-detection-crossroad-yolov3-1020¶
Use Case and High-Level Description¶
This is a YOLO V3 network fine-tuned for Person/Vehicle/Bike detection for security surveillance applications. It works in a variety of scenes and weather/lighting conditions.
Yolo V3 is a real-time object detection model implemented with Keras* from this repository and converted to TensorFlow* framework.
This model was pre-trained on Common Objects in Context (COCO) dataset with 80 classes and then fine-tuned for Person/Vehicle/Bike detection.
Example¶
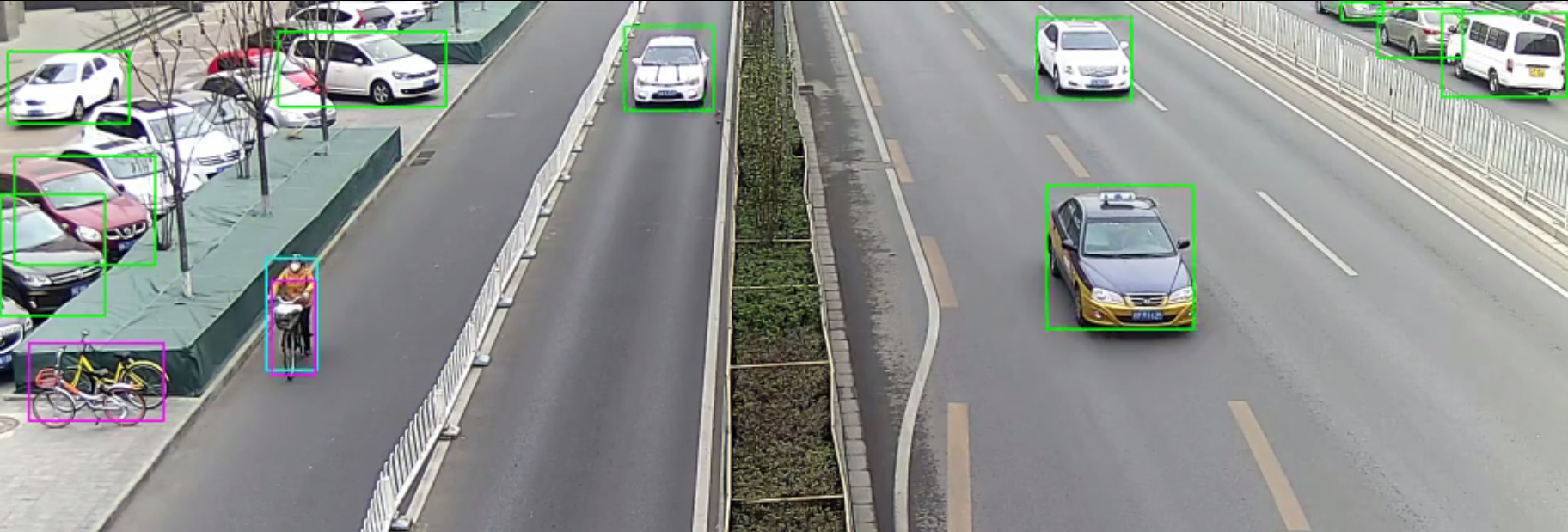
Specification¶
Metric |
Value |
---|---|
Mean Average Precision (mAP) |
48.89% |
AP people |
58.94% |
AP vehicles |
62.05% |
AP bikes/motorcycles |
25.66% |
GFlops |
65.98 |
MParams |
61.92 |
Source framework |
Keras* |
Average Precision (AP) is defined as an area under the precision/recall curve.
Validation dataset consists of 34757 images from various scenes and includes:
Type of object |
Number of bounding boxes |
---|---|
Vehicle |
229503 |
Pedestrian |
240009 |
Bike/Motorcycle |
62643 |
Similarly, training dataset has 17084 images with:
Type of object |
Number of bounding boxes |
---|---|
Vehicle |
121111 |
Pedestrian |
119546 |
Bike/Motorcycle |
30220 |
Inputs¶
Image, name: image_input
, shape: 1, 416, 416, 3
in the format B, H, W, C
, where:
B
- batch sizeH
- image heightW
- image widthC
- number of channels
Expected color order: BGR
.
Outputs¶
The array of detection summary info, name:
conv2d_58/Conv2D/YoloRegion
, shape:1, 255, 13, 13
. The anchor values are116,90, 156,198, 373,326
.The array of detection summary info, name:
conv2d_66/Conv2D/YoloRegion
, shape:1, 255, 26, 26
. The anchor values are30,61, 62,45, 59,119
.The array of detection summary info, name:
conv2d_74/Conv2D/YoloRegion
, shape:1, 255, 52, 52
. The anchor values are10,13, 16,30, 33,23
.
For each of the arrays the output format is B, N\*85, Cx, Cy
, where:
B
- batch sizeCx
,Cy
- cell indexN
- number of detection boxes for cell
Detection box has format [x
, y
, h
, w
, box_score
, class_no_1
, …, class_no_80
], where:
(
x
,y
) - coordinates of box center relative to the cellh
,w
- raw height and width of box, apply exponential function and multiply them by the corresponding anchors to get the absolute height and width valuesbox_score
- confidence of detection box in [0, 1] rangeclass_no_1
, …,class_no_80
- probability distribution over the classes in the [0, 1] range, multiply them by the confidence valuebox_score
to get confidence of each class
Since the model is fine-tuned on person/vehicle/bike detection dataset, it returns non-zero scores for the following classes:
person - the first class score
non-vehicle (bike/motorcycle) - the second class score
vehicle - the third class score Note that the indexes of these 3 classes are aligned with the indexes of the classes
person
,bike
, andcar
in the original Common Objects in Context (COCO) dataset. Also note that the model returns class scores for all 80 COCO classes for backward compatibility with the original Yolo V3.
Demo usage¶
The model can be used in the following demos provided by the Open Model Zoo to show its capabilities:
Legal information¶
[*] Other names and brands may be claimed as the property of others.