Hello Image Segmentation¶
This tutorial is also available as a Jupyter notebook that can be cloned directly from GitHub. See the installation guide for instructions to run this tutorial locally on Windows, Linux or macOS. To run without installing anything, click the launch binder button.
A very basic introduction to using segmentation models with OpenVINO™.
In this tutorial, a pre-trained road-segmentation-adas-0001 model from the Open Model Zoo is used. ADAS stands for Advanced Driver Assistance Services. The model recognizes four classes: background, road, curb and mark.
Imports¶
import cv2
import matplotlib.pyplot as plt
import numpy as np
import sys
from openvino.runtime import Core
sys.path.append("../utils")
from notebook_utils import segmentation_map_to_image
Load the Model¶
ie = Core()
model = ie.read_model(model="model/road-segmentation-adas-0001.xml")
compiled_model = ie.compile_model(model=model, device_name="CPU")
input_layer_ir = compiled_model.input(0)
output_layer_ir = compiled_model.output(0)
Load an Image¶
A sample image from the Mapillary Vistas dataset is provided.
# The segmentation network expects images in BGR format.
image = cv2.imread("../data/image/empty_road_mapillary.jpg")
rgb_image = cv2.cvtColor(image, cv2.COLOR_BGR2RGB)
image_h, image_w, _ = image.shape
# N,C,H,W = batch size, number of channels, height, width.
N, C, H, W = input_layer_ir.shape
# OpenCV resize expects the destination size as (width, height).
resized_image = cv2.resize(image, (W, H))
# Reshape to the network input shape.
input_image = np.expand_dims(
resized_image.transpose(2, 0, 1), 0
)
plt.imshow(rgb_image)
<matplotlib.image.AxesImage at 0x7f9fd47f0fd0>
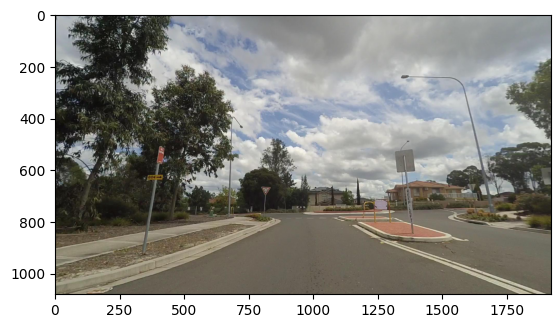
Do Inference¶
# Run the inference.
result = compiled_model([input_image])[output_layer_ir]
# Prepare data for visualization.
segmentation_mask = np.argmax(result, axis=1)
plt.imshow(segmentation_mask.transpose(1, 2, 0))
<matplotlib.image.AxesImage at 0x7f9fd46df0a0>
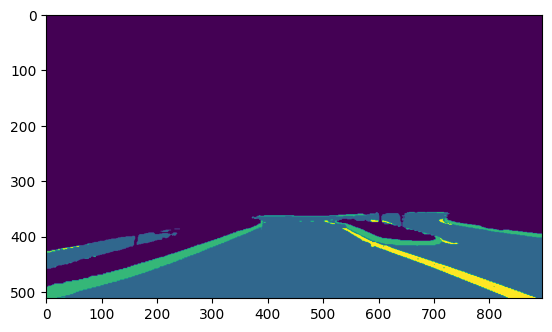
Prepare Data for Visualization¶
# Define colormap, each color represents a class.
colormap = np.array([[68, 1, 84], [48, 103, 141], [53, 183, 120], [199, 216, 52]])
# Define the transparency of the segmentation mask on the photo.
alpha = 0.3
# Use function from notebook_utils.py to transform mask to an RGB image.
mask = segmentation_map_to_image(segmentation_mask, colormap)
resized_mask = cv2.resize(mask, (image_w, image_h))
# Create an image with mask.
image_with_mask = cv2.addWeighted(resized_mask, alpha, rgb_image, 1 - alpha, 0)
Visualize data¶
# Define titles with images.
data = {"Base Photo": rgb_image, "Segmentation": mask, "Masked Photo": image_with_mask}
# Create a subplot to visualize images.
fig, axs = plt.subplots(1, len(data.items()), figsize=(15, 10))
# Fill the subplot.
for ax, (name, image) in zip(axs, data.items()):
ax.axis('off')
ax.set_title(name)
ax.imshow(image)
# Display an image.
plt.show(fig)
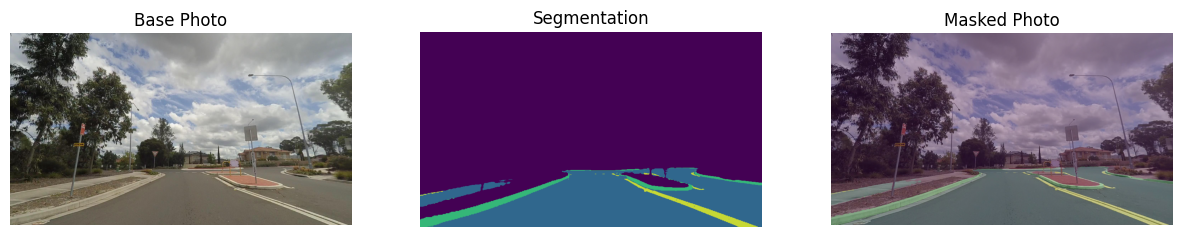