vehicle-license-plate-detection-barrier-0123¶
Use Case and High-level Description¶
This is a MobileNetV2 + SSD-based vehicle and (Chinese) license plate detector for the “Barrier” use case.
Example¶
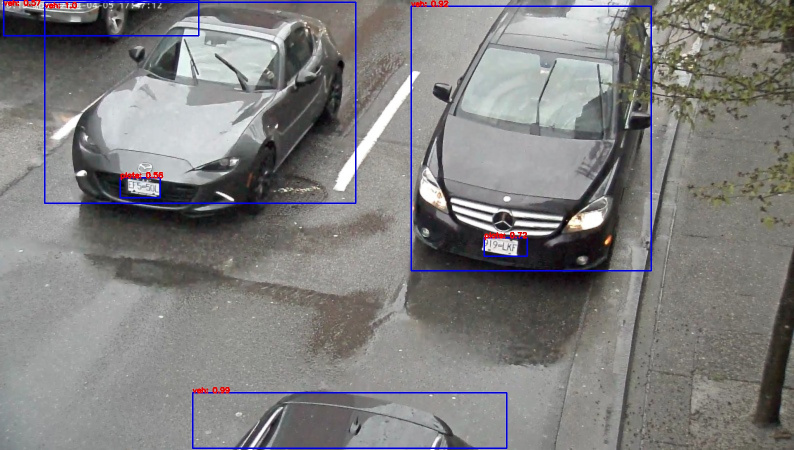
Specification¶
Metric |
Value |
---|---|
Mean Average Precision (mAP) |
99.52% |
AP vehicles |
99.90% |
AP plates |
99.13% |
Car pose |
Front facing cars |
Min plate width |
96 pixels |
Max objects to detect |
200 |
GFlops |
0.271 |
MParams |
0.547 |
Source framework |
TensorFlow* |
Average Precision (AP) is defined as an area under the precision/recall curve. Validation dataset is BIT-Vehicle.
Input¶
Original Model¶
An input image, name: input
, shape: 1, 256, 256, 3
, format: B, H, W, C
, where:
B
- batch sizeH
- image heightW
- image widthC
- number of channels
Expected color order: RGB
. Mean values: [127.5, 127.5, 127.5], scale factor for each channel: 127.5
Converted Model¶
An input image, name: input
, shape: 1, 3, 256, 256
, format B, C, H, W
, where:
B
- batch sizeC
- number of channelsH
- image heightW
- image width
Expected color order is BGR
.
Output¶
Original Model¶
The net outputs a blob with the shape: 1, 1, 200, 7
in the format 1, 1, N, 7
, where N
is the number of detected bounding boxes. For each detection, the description has the format: [image_id
, label
, conf
, x_min
, y_min
, x_max
, y_max
], where:
image_id
- ID of the image in the batchlabel
- predicted class IDconf
- confidence for the predicted class(
x_min
,y_min
) - coordinates of the top left bounding box corner(
x_max
,y_max
) - coordinates of the bottom right bounding box corner.
Converted Model¶
The net outputs a blob with the shape: 1, 1, 200, 7
in the format 1, 1, N, 7
, where N
is the number of detected bounding boxes. For each detection, the description has the format: [image_id
, label
, conf
, x_min
, y_min
, x_max
, y_max
], where:
image_id
- ID of the image in the batchlabel
- predicted class IDconf
- confidence for the predicted class(
x_min
,y_min
) - coordinates of the top left bounding box corner(
x_max
,y_max
) - coordinates of the bottom right bounding box corner.
Download a Model and Convert it into Inference Engine Format¶
You can download models and if necessary convert them into Inference Engine format using the Model Downloader and other automation tools as shown in the examples below.
An example of using the Model Downloader:
python3 <omz_dir>/tools/downloader/downloader.py --name <model_name>
An example of using the Model Converter:
python3 <omz_dir>/tools/downloader/converter.py --name <model_name>
Legal Information¶
The original model is distributed under the Apache License, Version 2.0. A copy of the license is provided in <omz_dir>/models/public/licenses/APACHE-2.0.txt
.
[*] Other names and brands may be claimed as the property of others.